[Seminar] "Dynamics and Learning - Future of AI with Robotics Applications" by Mr. Motoya Ohnishi
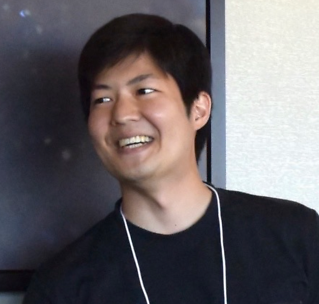
Date
Location
Description
Dear all,
Neural Computation Unit (Doya Unit) would like to invite you to a seminar as follows.
Speaker: Mr. Motoya Ohnishi
Website: https://homes.cs.washington.edu/~mohnishi/
Zoom Information:
https://oist.zoom.us/j/94407449683?pwd=eEFYVHF6WmwxNnpEV3E0Sm1mSVAzdz09
Meeting ID: 944 0744 9683
Passcode: 751190
Title: Dynamics and Learning - Future of AI with Robotics Applications
Abstract: With the increasing demands on AI robotics technology, there has been a surge of interest in merging machine learning ー a data-driven approach based on statistics ー, and dynamical systems or control theory, which deals with dynamics.
This seminar primarily revolves around a discussion of my research endeavors in reinforcement learning (RL) and its extensions, conducted within academia and at several companies.
Initially, I will provide a succinct overview of our RL algorithm, leveraging a recent control theoretical tool as an oracle to theoretically ensure learning efficiency. Subsequently, employing a novel decision-making formulation with the Koopman operator, I will illustrate the realization of a diverse array of dynamic behaviors.
Furthermore, an additional highlight of our exploration involves the successful extension of RL beyond its conventional reliance on the Bellman equation, encompassing dynamic programming across entire paths. The new framework has proven beneficial in addressing challenges related to path following.
On the other hands, a machine learning algorithm itself can be seen as a dynamical system, and this perspective has a huge theoretical and practical potential. An illustrative example from my body of work will underscore the practical implications of adopting this insightful perspective.
Finally, I aim to delve into potential future trajectories for AI research through the lens of dynamical systems. Furthermore, I would also like to discuss a recent progress on end-to-end learning of robotics problems with a foundation model.
We hope to see many of you at the seminar.
Sincerely,
Neural Computation Unit
Contact: ncus@oist.jp
Subscribe to the OIST Calendar: Right-click to download, then open in your calendar application.