[Seminar] "A Recurrence-based Direct Method for Stability Analysis" by Mr. Roy Siegelmann
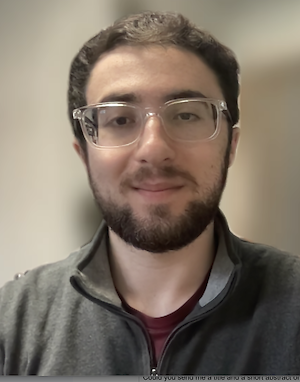
Date
Location
Description
Dear all,
Neural Computation Unit (Doya Unit) would like to invite you to a seminar as follows.
Speaker: Mr. Roy Siegelmann (the Mallada Laboratory, Johns Hopkins Whiting School of Engineering )
Website: https://mallada.ece.jhu.edu
Zoom Information:
https://oist.zoom.us/j/94407449683?pwd=eEFYVHF6WmwxNnpEV3E0Sm1mSVAzdz09
Meeting ID: 944 0744 9683
Passcode: 751190
Title: A Recurrence-based Direct Method for Stability Analysis
Abstract: Incorporating neural memory models based on attractor dynamics into neural networks has been conjectured to keep the computational network smaller and more practical to train. However, state-of-the-art memory models are not scalable in terms of encompassing richer and larger memories. At the crux of this is the dependence of neural models is the reliance on existence of a Lyapunov function, a function whose value monotonically decreases along the trajectories of the dynamical system. Unfortunately, finding a Lyapunov function is often tricky and requires ingenuity, domain knowledge, or significant computational power. At the core of this challenge is the fact that the method requires every sub-level set to be forward invariant, thus implicitly coupling the geometry of the function and the trajectories of the system. We seek to disentangle this dependence by developing a direct method that substitutes the concept of invariance with the more flexible notion of recurrence. We show that, under mild conditions, the recurrence of sub-level sets is sufficient to guarantee stability and introduce the appropriate stronger notions to obtain asymptotic stability and exponential stability, which in turn may enable new types of memory model.
We hope to see many of you at the seminar.
Sincerely,
Neural Computation Unit
Contact: ncus@oist.jp
Subscribe to the OIST Calendar: Right-click to download, then open in your calendar application.