[Seminar] "Multiple independent goal-directed learning for emergent adaptive behaviour in robots" by Dr. Danish Shaikh
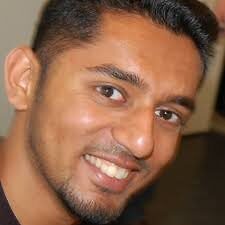
Date
Location
Description
Dear all,
Neural Computation Unit (Doya Unit) would like to invite you to a seminar as follows.
Date: Wedenesday, November 20, 2019
Time: 10:00 – 11:00
Venue: Meeting room C016, Lab1 Bldg.
Speaker: Dr. Danish Shaikh
University of Southern Denmark Embodied Systems for Robotics and Learning, The Maersk Mc-Kinney Moller Institute
Title:
Multiple independent goal-directed learning for emergent adaptive behaviour in robots
Abstract:
Model-free robot controllers are typically designed using black box approaches such as machine learning algorithms. Machine learning has been successfully applied to robotic perception and motor control, and recently deep learning has allowed end-to-end learning of low-level motor commands directly from high-dimensional, raw multi-sensor data. In the biological brain various regions use various training inputs (multiple sensory signals, efferent motor signals, and other intermediate signals such as dopamine signalling) to learn across different spatial and temporal scales, utilising a combination of structural, synaptic and non-synaptic plasticity rules. From a robotics perspective, this implies that different robot behaviours typically require different sets of multi-modal inputs and must learn to adapt their parameters from them. This is non-trivial and makes designing robot controllers challenging.
In this talk I will present the paradigm of multiple independent goal-directed learning, where the idea is that intelligent, adaptive behaviour can emerge from interaction between multiple, independent task-specific machine learning algorithms. I will present my preliminary simulation work [1,2] on applying this approach to goal-directed mobile robot navigation, where multiple plasticity rules, running independently and in parallel, tune context-dependent and task-specific parameters within the robot’s controller via interaction with the environment.
References:
[1] Shaikh, D., Manoonpong, P., “A neuroplasticity-inspired neural circuit for acoustic navigation with obstacle avoidance that learns smooth motion paths”, in Neural Computing and Applications, 31(6), 1765-1781, 2019, doi: 10.1007/s00521-018-3845-y
[2] Shaikh, D., Manoonpong, P., “A Neural Circuit for Acoustic Navigation combining Heterosynaptic and Non-synaptic Plasticity that learns Stable Trajectories”, in Engineering Applications of Neural Networks: 18th International Conference, EANN 2017, Athens, Greece, August 25–27, 2017, Proceedings, Boracchi, G., Iliadis L., Jayne C. , Likas A., Eds., Springer, Cham, Communications in Computer and Information Science, vol. 744, pp. 544–555, 2017, doi: 10.1007/978-3-319-65172-9_46
We hope to see many of you at the seminar.
Sincerely,
Neural Computation Unit
Contact: ncus@oist.jp
Intra-Group Category
Subscribe to the OIST Calendar: Right-click to download, then open in your calendar application.