[Seminar] "Critical Brains for Autonomous Robots" by Dr. Herrmann
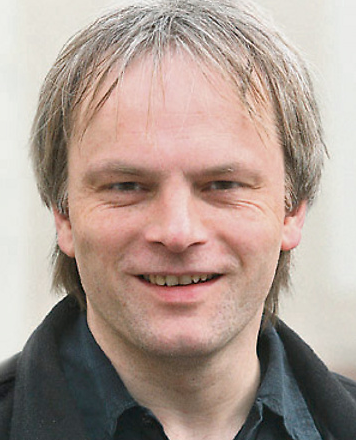
Date
Location
Description
Dear all,
Neural Computation Unit (Doya Unit) would like to invite you to a seminar as follows.
--
Date: Tuesday, April 24
Time: 11:00-12:00
Venue: Meeting Room D015 - Lab1 Bldg
Speaker: Dr. J. Michael Herrmann
Institute for Perception, Action and Behavior, School of Informatics, University of Edinburgh and Edinburgh Centre for Robotics, Edinburgh, Scotland, United Kingdom
Title: Critical Brains for Autonomous Robots
Abstract: Self-Organized criticality, according to Per Bak (1996), describes the way how nature works. The reasons for the frequent occurrence of criticality can be seen in the resulting benefits for an evolving or artificial system which include maximal sensitivity, optimal dynamic range and the richness of resulting behaviors. Criticality is found in neural systems and can be applied also in the design of algorithms for optimization and robot control. After considering a number of examples from previous work on neural avalanches, particle swarms and the generation of behavior in autonomous robots, we will address the question how we can decide whether a system is critical and how a given system can be controlled towards criticality. In this way, we will be able to identify relations among different approaches to criticality and methods to combine self-organized critical exploratory activity and goal-directed behavior.
Dr. Herrmann will also give a talk on Wednesday 25th from 11 am at meeting room C015 Lab1 about the specific topic below:
Title: W. Ross Ashby's Homeostat
Abstract: Ashby's Homeostat performs a controlled random search in the parameter space of an essentially linear system, not unlike recent evolutionary algorithms. In this way, it achieves a stabilization of dynamical system after strong parametric perturbations and is thus a model system for Ashby's concept of ultrastability. Because the return to the "viability zone" of the system is achieved in large systems only after exponentially long transients, we consider an adaptive variant, the "Learning Homeostat", where the parameters are readjusted by a gradient dynamics with the effect that the transient period becomes virtually independent on the dimension. The system bears some interest because it provides an example of a learning mechanism with a parameter dynamics on the same time scale as the state evolution, it is analytically solvable and has implications for control mechanisms in natural or artificial embedded agents.
We hope to see many of you at the seminar.
Sincerely,
Kikuko Matsuo
Neural Computation Unit
Intra-Group Category
Subscribe to the OIST Calendar: Right-click to download, then open in your calendar application.