[Seminar] “On the way to the automated discovery of novel patterns and dynamics in physical and chemical systems by intrinsically motivated deep learning. ” by Dr. Reinke
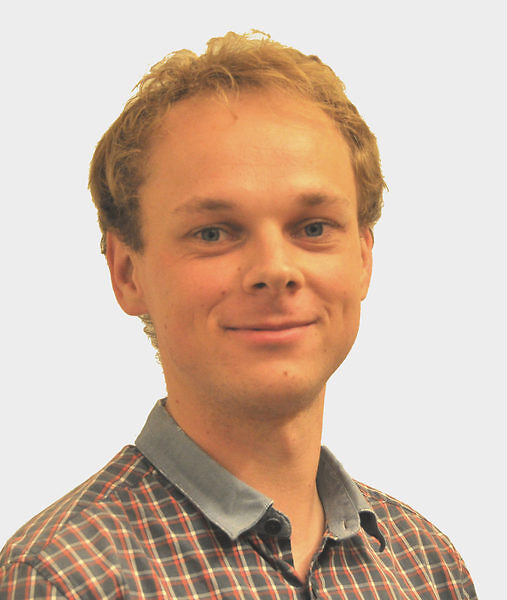
Date
Location
Description
Dear all,
Neural Computation Unit (Doya Unit) would like to invite you to a seminar as follows.
Date: Thursday, May 30, 2019
Time: 14:00 – 15:00
Venue: Seminar room B503, Lab1 Bldg.
Speaker: Chris Reinke
Flowers Lab, Inria Bordeaux, France
Title:
On the way to the automated discovery of novel patterns and dynamics in physical and chemical systems by intrinsically motivated deep learning.
Abstract:
Many physical and chemical systems that we encounter are complex and dynamic. For example, the air flow around a rotor blade of a wind turbine might form different turbulence patterns over time. Those dynamics depend on parameters such as the form of the rotator blade, wind direction and others. To understand such systems we need to explore which dynamics and patterns can be produced. Unfortunately, many experiments might be needed to uncover those dynamics, which if manually done are often very time intensive. A solution comes from the application of AI and robotics, which perform and control the exploration of such systems automatically.
We hope to see many of you at the seminar.
Sincerely,
Neural Computation Unit
Contact: ncus@oist.jp
Intra-Group Category
Subscribe to the OIST Calendar: Right-click to download, then open in your calendar application.