Deep Reinforcement Learning by Parallelizing Reward and Punishment using the MaxPain Architecture, Dr. Jiexin Wang
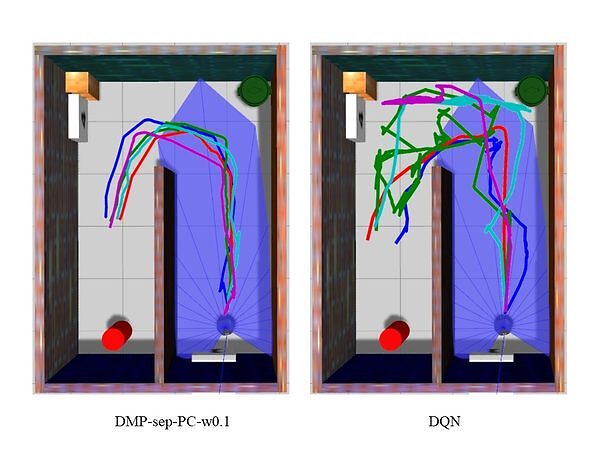
Date
Description
Neural Computation Unit (Doya Unit) would like to invite you to a seminar as follows.
--
Date: Wednesday, November 14
Time: 13:00-
Venue: Meeting Room D015 - L1 Bldg
Speaker: Dr. Jiexin Wang, a researcher at the department of Brain Robot Interface, ATR Computational Neuroscience Laboratories.
Title: Deep Reinforcement Learning by Parallelizing Reward and Punishment using the MaxPain Architecture
Abstract: Traditionally, reinforcement learning treats punishments as negative rewards. However, in biological decision systems, some evidence shows that animals have separate systems for rewards and punishments. The MaxPain architecture parallelizes the predictions of rewards and punishments and scales them into dual-attribute policies, and has been shown to both improve the learning speed and the learning of safer behaviors. This paper extends the MaxPain architecture into a deep reinforcement learning framework using convolutional neural networks to approximate two action-value functions. To derive the behavioral policy, we consider the mixture distributions of the policies computed from the two action-value functions. For evaluation, we compare the MaxPain architecture with count-based exploration and a reward-decomposing structure called Hybrid Reward Architecture (HRA) in grid-world navigation and vision-based navigation in a U-shape maze in the Gazebo robot simulation environment. The simulation results show the superiority of the MaxPain approach over the count- based method because the MaxPain agents efficiently avoid dead-end states by predicting future punishments. In addition, the MaxPain agents learn safe behaviors, while the HRA agents learn similar behaviors, as in the case of no punishments.
--
We hope to see many of you.
Sincerely,
ncus@oist.jp
Emiko Asato
Neural Computation Unit
Subscribe to the OIST Calendar: Right-click to download, then open in your calendar application.