[PhD Thesis Presentation_Zoom] ‐ Evropi Toulkeridou – “Automated segmentation of micro-CT images by deep learning and its application to comparative morphology”
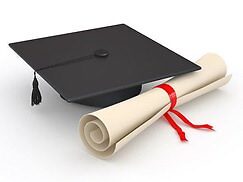
Date
Location
Description
Presenter: Evropi Toulkeridou
Supervisor: Prof. Evan P Economo
Co-supervisor: Prof. Kenji Doya Supervisor
Unit: Biodiversity and Biocomlexity Unit
Title: Automated segmentation of micro-CT images by deep learning and its application to comparative morphology
Abstract:
Semantic segmentation, i.e., association of each pixel of an image with a component label, is one of the most fascinating but challenging problems in the field of computer vision. During semantic segmentation, the image is divided into non-overlapping areas which are subsequently clustered together if found that they belong to the same object. A natural field of potential application is in organismal biology, where researchers are increasingly using three-dimensional (3D) scanning (e.g., confocal scanning or micro-computed tomography, micro-CT) which produces data-rich volumetric images for precise and comprehensive anatomical characterization.
To date, the segmentation of anatomical structures remains a big bottleneck to research, as it is commonly performed with highly tedious and time-consuming manual work. During recent years, however, machine learning (ML) methods are an emerging approach to overcoming this limitation, especially with the use of deep learning techniques such as convolutional neural networks (CNNs), which proved to be very efficient and, as such, promising candidates for image segmentation.
The main objective of this PhD project was to develop a pipeline for the fully-automated segmentation of anatomical structures in micro-CT images of insects using state-of-the-art deep learning methods. The restricted number of high-resolution 3D labeled images available necessitated the use of a CNN architecture that performs segmentation satisfactorily even with limited data; the U-Net architecture is such a convolutional network that has shown good performance in medical images using few annotated images.
Ant brains were selected for a test case, and a dataset was assembled of semi-manually segmented brain images of 94 ant species. It should be noted that both automated classification and segmentation tasks typically require big datasets for training and validation, which can be a challenge for researchers to produce for any given application. Since no dataset of micro-CT images of ant brains existed for the current case study, a new extensive dataset was created across a wide variation of ants. Its existence can be of great importance, as brain images of ants are not dissimilar to those of other insects; therefore, our dataset can act as a starting point for the development of an even bigger library of micro-CT images of insects, and work as a pre-training dataset for future CNNs.
The chosen species set was designed to be interesting for further evolutionary morphology analysis. In the second part of the study, we tested the social brain hypothesis for ants, i.e., the question of whether there is a connection on the brain investment and the sociality of the species. Further volumetric statistical analysis was performed combining the phylogenetic data of the species, leading to the rejection of the hypothesis.
Finally, it should be noted that our network is generalizable for segmenting the whole neural system in full-body scans, and works in tests on distantly related and morphologically divergent insects (e.g., fruit flies). The latter suggests algorithms such as our network can be applied generally across diverse taxa
Intra-Group Category
Subscribe to the OIST Calendar: Right-click to download, then open in your calendar application.