[PhD Thesis Presentation] ‐ Mr. Tadashi Kozuno "Efficient and Noise-Tolerant Reinforcement Learning Algorithms via Theoretical Analysis of Gap-Increasing and Softmax Operators"
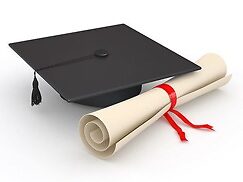
Date
Location
Description
Presenter: Mr. Kozuno Tadashi
Supervisor: Professor Kenji Doya
Unit: Neural Computation Unit
Title: Efficient and Noise-Tolerant Reinforcement Learning Algorithms via Theoretical Analysis of Gap-Increasing and Softmax Operators
ABSTRACT:
Model-free deep Reinforcement Learning (RL) algorithms, a combination of deep learning and model-free RL algorithms, have attained remarkable successes in solving complex tasks such as video games. However, theoretical analyses and recent empirical results indicate its proneness to various types of value update errors including but not limited to estimation error of updates due to finite samples and function approximation error. Because real-world tasks are inherently complex and stochastic, such errors are inevitable, and thus, the development of error-tolerant RL algorithms are of great importance for applications of RL to real problems. To this end, I propose two error-tolerant algorithms for RL called Conservative Value Iteration (CVI) and Gap-increasing RetrAce for Policy Evaluation (GRAPE).
CVI unifies value-iteration-like single-stage-lookahead algorithms such as soft value iteration, advantage learning and Ψ-learning, all of which are characterized by the use of a gap-increasing operator and/or softmax operator in value updates. We provide detailed theoretical analysis of CVI that not only shows CVI’s advantages but also contributes to the theory of RL in the following two points: First, it elucidates pros and cons of gap-increasing and softmax operators. Second, it provides an actual example in which performance of algorithms with max operator is worse than that of algorithms with soft- max operator demonstrating the limitation of traditional greedy value updates.
GRAPE is a policy evaluation algorithm extending advantage learning (AL) and re- trace, both of which have different advantages: AL is noise-tolerant as shown through our theoretical analysis of CVI, while retrace is efficient in that it is off-policy and allows the control of bias-variance trade-off. Theoretical analysis of GRAPE shows that it enjoys the merits of both algorithms. In experiments, we demonstrate the benefit of GRAPE combined with a variant of trust region policy optimization and its superiority to previous algorithms.
Intra-Group Category
Subscribe to the OIST Calendar: Right-click to download, then open in your calendar application.