OCNC2010
Okinawa Computational Neuroscience Course 2010
June 14 - July 1, 2010 in Okinawa, Japan
Okinawa Computational Neuroscience Course 2010
The aim of the Okinawa Computational Neuroscience Course is to provide opportunities for young researchers with theoretical backgrounds to learn the latest advances in neuroscience, and for those with experimental backgrounds to have hands-on experience in computational modeling.
We invite graduate students and postgraduate researchers to participate in the course, held from June 15th through July 2nd at an oceanfront seminar house of the Okinawa Institute of Science and Technology.
In 2004-2006, OCNC focused on the brain's computation at different levels: Bayesian computation by neural populations (2004), learning and prediction for behavior (2005), single neurons as computational devices (2006). In 2007, the course included a wide array of topics: single neurons, networks, and behaviors.
In 2008-2010, the course will run for three weeks with ample time in the afternoon for student projects. The first week will be devoted to methods only, with introductory talks in the morning and software tutorials in the afternoon.
The sponsor will provide lodging and meals during the course and support travel for those without funding. We hope that this course will be a good opportunity for theoretical and experimental neuroscientists to meet each other and to explore the attractive nature and culture of Okinawa, the southernmost island prefecture of Japan.
Schedule
A list of lecture topics can be found at the program page.
- January 4:
- Application process opens
- February 15:
- Application process closes
- Early March:
- Notification of acceptance
- Mid March:
- Confirmation of acceptance
- June 13:
- Arrival
- June 14-19:
- Methods
- June 21-26:
- Neurons, Networks and Behaviors I
- June 27-July 1:
- Neurons, Networks and Behaviors II
- July 2:
- Departure
Organizers
- Erik DeSchutter
- Kenji Doya
- Klaus Stiefel
- Jeff Wickens
Lecturers
- Amari, Shun-Ichi
- Arbuthnott, Gordon
- Brette, Romain
- Dayan, Peter
- De Schutter, Erik
- Doya, Kenji
- Gutkin, Boris
- Hellgren Kotaleski, Jeanette
- Izhikevich, Eugene
- Karpova, Alla
- Kawato, Mitsuo
- Knöpfel, Thomas
- Obermayer, Klaus
- Ohzawa, Izumi
- Sharpee, Tatyana
- Spruston, Nelson
- Stiefel, Klaus
Tutors
- Akemann, Walther
- Gunay, Cengiz
- Le Franc, Yann
- Potjans, Wiebke
- Potjans, Tobias
- Torben-Nielsen, Ben
- Wang, Ping
- van der Meer, Matthijs (Matt)
Neural Microcircuitry Conference
Neural Microcircuitry Conference
Interested students will be able to attend evening and Sunday sessions of this conference, separate registration required.
All lectures take place in the Seminar Room, OIST Seaside House unless otherwise indicated.
Week 1: Methods (Jun 14-19)
Monday, June 14
- 9:15-9:30
- Introduction by the course organizers
- 9:30-10:30
- Opening Lecture: Brain, Information and Mathematical Neuroscience (Shun-Ichi Amari)
- 11:00-12:30
- Parallel track:
Biologists: Introduction to numerical methods for ordinary and partial differential equations (Kenji Doya)
Theoreticians: Neurobiology for Mathematicians (Meeting Room 1) (Gordon Arbuthnott)
- 14:00-16:00
- Student poster presentations I (Abiva-Kanitscheider)
- 16:00-18:00
- Student poster presentations II (Kubánek-You)
- 19:00-21:00
- Reception
Tuesday, June 15
- 09:30-12:30
- Introduction to modeling voltage-gated channels and interpretation of imaging data (Thomas Knöpfel)
- 14:00-15:00
- Introduction of the Tutors
- 15:00-16:00
- A broad overview of the different simulation environments and modeling tools available
- 16:30-18:30
- Python tutorial
Wednesday, June 16
- 09:30-12:30
- Introduction to modeling morphologically detailed neurons (Klaus Stiefel)
- 14:00-16:00
- NEURON tutorial
- 17:00-18:00
- Matlab tutorial
Thursday, June 17
- 09:30-12:30
- Introduction to integrate-and-fire models (Romain Brette)
- 14:00-16:00
- NEST tutorial
- 16:30-18:30
- Advanced NEURON tutorial
Friday, June 18
- 09:30-12:30
- Introduction to modeling biochemical reactions, diffusion and reaction-diffusion systems (Erik De Schutter)
- 14:00-16:00
- STEPS tutorial or meeting with Dr. Brette or Dr. Knöpfel
- 16:30-17:30
- High performance computing tutorial
- 17:30-18:30
- Good modeling practice tutorial
Saturday, June 19
- 09:30-12:30
- Introduction to reinforcement learning and Bayesian inference (Kenji Doya)
- 14:00-18:00
- Project work
Week 2: Neurons, Networks and Behavior I (Jun 21-26)
Monday, June 21
- 09:30-12:30
- Microcircuits involved in motion – insights gained through modeling (Jeanette Hellgren Kotaleski)
- 14:00-16:00
- Project work or meeting with Dr. De Schutter
- 16:00-18:00
- Project work
Tuesday, June 22
- 09:30-12:30
- Mechanisms of dendritic integration and plasticity (Nelson Spruston)
- 14:00-16:00
- Project work, or visit to Lab Building 1, or meeting with Dr. Doya or Dr. Stiefel
- 16:00-18:00
- Project work
Wednesday, June 23
- 09:30-12:30
- Psychological and Neural Reinforcement Learning (Peter Dayan)
- 14:00-16:00
- Project work or meeting with Dr. Kotaleski or Dr. Spruston
- 16:00-18:00
- Visit to Lab Building 1
Thursday, June 24
- 09:30-12:30
- Cerebellar internal model, Purkinje cell LTD as supervised learning rule supported by bioinformatics, and control of learning degree-of-freedom by electrical coupling in Inferior Olive nucleus (Mitsuo Kawato)
- 14:00-16:00
- Project work or meeting with Dr. Dayan
- 16:00-18:00
- Project work
Friday, June 25
- 09:30-12:30
- Extending the notion of receptive field for studying high-order visual neurons (Izumi Ohzawa)
- 14:00-16:00
- Project work or meeting with Dr. Kawato
- 16:00-18:00
- Project work
Saturday, June 26
- 09:30-12:30
- Network Models of Primary Visual Cortex (Klaus Obermayer)
- 14:00-18:00
- Project work
Week 3: Neurons, Networks and Behavior II (Jun 28-Jul 1)
Monday, June 28
- 09:30-12:30
- Technologies for in vivo circuit research (Alla Karpova)
- 14:00-16:00
- Project work or meeting with Dr. Obermayer
- 16:00-18:00
- Project work
Tuesday, June 29
- 09:30-12:30
- Large-scale modeling of the brain (Eugene Izhikevich)
- 14:00-16:00
- Project work or meeting with Dr. Karpova or Dr. Izhikevich
- 16:00-18:00
- Project work
Wednesday, June 30
- 09:30-12:30
- Maximally informative irregularities in neural circuits (Tatyana Sharpee)
- 14:00-16:00
- Project work or meeting with Dr. Sharpee or Dr. Gutkin
- 16:00-18:00
- Project work
Thursday, July 1
- 09:30-12:30
- Theoretical approaches to dynamics of neuronal excitability: spike generation, neuromodulation, synchrony and oscillatory dendrites (Boris Gutkin)
- 14:00-18:00
- Student project presentations
- 19:00-21:00
- Banquet
Lecture Abstracts & Readings
Shun-ichi Amari
Opening Lecture: Brain, Information and Mathematical Neuroscience
The brain consists of a huge number of mutually connected neurons. We need to study molecular and cellular basis of functions of neurons as well as networks or systems level functions. The brain is made of living materials, which are very special materials. Their functions are mostly information processing and memory formation. Further our mind has emerged and realized by using those materials and their functions. We will overview a history of evolution of the universe, showing how life has emerged from materials, what is the principal role of life and information in it, as well as the role of mind.
Theoretical neuroscience, in particular mathematical neuroscience, is one of the very important constituents of the brain research, although it has not yet been completely established. I will discuss its methods and scopes, introducing principles of mathematical neuroscience.
Gordon Arbuthnott
NEUROBIOLOGY FOR MATHEMATICIANS…
The usual accusation of 'unreasonable naivety', which is often presented to computational scientists by experimental biologists, is sometimes only a cover for their own ignorance of computational methods.
On the other hand, just because biology is complicated is no reason not to try to understand it! Perhaps the best format for this session would be a question and answer one. Not that I will have all the answers but at least some of the systems biology of the nervous system is within my ken and we can go through several of the brain areas that interest students in this year's course.
In the past I've used 'Brain Architecture' by Larry Swanson as a backbone to talk about order in the chaos of neuroanatomy but there is no reason to stick to the text if you would like to talk about other things. In particular there are very few details on synaptic relationships in Swanson's book. In fact there are very few details….
One recurring theme will inevitably be that many of the quantitative answers to questions that interest you – are extraordinarily hard to come by. We can cover why that is too!
Lets see what we can learn from each other in the time!
Gordon.
Suggested reading
Brain Architecture
Swanson. LA
OUP 2003
Romain Brette
Introduction to integrate-and-fire models
This lecture will focus on phenomenological descriptions of neurons known as "integrate-and-fire models", which are convenient analytical and numerical tools to understand neural function. In the first part of the lecture, I will show why these are reasonable neuron models, how to relate them with biophysical descriptions of neurons and how to model various electrophysiological properties (e.g. adaptation). I will then present the general properties of these models: coincidence detection vs. integration, balanced vs. oscillator regime, reliability of spike timing, irregularity of interspike intervals. In the second part of the lecture, I will introduce a few concepts of network dynamics and basic elements of synaptic plasticity models. The concepts will be illustrated with concrete computational examples using the Brian simulator.
Suggested reading
- Wulfram Gerstner and Werner M. Kistler: Spiking Neuron Models. Single Neurons, Populations, Plasticity. Cambridge University Press (August 2002)
- Peter Dayan and L.F. Abbott.: Theoretical Neuroscience: Computational And Mathematical Modeling of Neural Systems. MIT Press.
Peter Dayan
Psychological and Neural Reinforcement Learning
We will expand on Kenji Doya's description of computational and neural aspects of reinforcement learning. We will review four systems involved in control: three instrumental decision-makers (model-based, model-free and episodic); and one Pavlovian controller. We will consider computational, psychological and neural aspects of each, and the ways that they can cooperate and compete. We will consider additional factors in the models such as prior expectations, uncertainty and exploration, and also model-free and model-based routes to psychiatric conditions such as depression.
Suggested reading
- Rangel A, Camerer C & Montague PR: A framework for studying the neurobiology of value-based decision making. Nature Reviews Neuroscience 9(7):545 (2008)
http://www.ncbi.nlm.nih.gov/pubmed/18545266http://www.ncbi.nlm.nih.gov/pubmed/18545266 - Daw, ND & Doya, K: The computational neurobiology of learning and reward. Current Opinion in Neurobiology 16(2):199. (2006)
http://www.ncbi.nlm.nih.gov/pubmed/16563737http://www.ncbi.nlm.nih.gov/pubmed/16563737 - Dayan P & Huys QJM: Serotonin, inhibition and negative mood. Public Library of Science: Computational Biology 4 e4. (2008)
http://www.gatsby.ucl.ac.uk/~dayan/papers/dayhuys08.pdfhttp://www.gatsby.ucl.ac.uk/~dayan/papers/dayhuys08.pdf - Huys QJM & Dayan P: A Bayesian formulation of behavioral control. Cognition 113 314-328 (2009)
http://www.gatsby.ucl.ac.uk/~dayan/papers/huysdayan09.pdfhttp://www.gatsby.ucl.ac.uk/~dayan/papers/huysdayan09.pdf - Dayan, P, Niv, Y, Seymour, BJ & Daw, ND: The misbehavior of value and the discipline of the will. Neural Networks 19 1153-1160. (2006)
http://www.gatsby.ucl.ac.uk/~dayan/papers/dayanetal2006.pdfhttp://www.gatsby.ucl.ac.uk/~dayan/papers/dayanetal2006.pdf - Daw, ND, Niv, Y & Dayan, P: Uncertainty-based competition between prefrontal and dorsolateral striatal systems for behavioral control. Nature Neuroscience 8 1704-1711. (2005)
http://www.gatsby.ucl.ac.uk/~dayan/papers/dawnivd05.pdfhttp://www.gatsby.ucl.ac.uk/~dayan/papers/dawnivd05.pdf - Dayan P: The role of value systems in decision making. In Engel C & Singer W, editors, Better than Conscious? Decision Making, the Human Mind, and Implications for Institutions Frankfurt, Germany: MIT Press, 51-70. (2008)
http://www.gatsby.ucl.ac.uk/~dayan/papers/dayval08.pdfhttp://www.gatsby.ucl.ac.uk/~dayan/papers/dayval08.pdf
Erik De Schutter
Modeling biochemical reactions, diffusion and reaction-diffusion systems
In my first talk I will use calcium dynamics modeling as a way to introduce deterministic solution methods for reaction-diffusion systems. The talk covers exponentially decaying calcium pools, diffusion, calcium buffers and buffered diffusion, and calcium pumps and exchangers. I will describe properties of buffered diffusion systems, ways to characterize them and new approximations that we developed for use in large neuron models.
In my second talk I will turn towards stochastic reaction-diffusion modeling.Two methods will be described: Gillespie's Stochastic Simulation algorithm extended to simulate diffusion and particle-based methods. I will discuss some of the problems in generating correct descriptions of microscopic 3D geometries and briefly describe the STEPS software. I will then describe two applications of stochastic reaction modeling of LTD induction in Purkinje cells and stochastic diffusion modeling of anomalous diffusion in spiny dendrites.
Suggested reading
- U.S. Bhalla and S. Wils: Reaction-diffusion modeling. In Computational Modeling Methods for Neuroscientists, E. De Schutter ed., MIT Press, Boston. 61–92 (2010)
- E. De Schutter: Modeling intracellular calcium dynamics. In Computational Modeling Methods for Neuroscientists, E. De Schutter ed., MIT Press, Boston. 61–92 (2010)
- S. Kuroda, N. Schweighofer and M. Kawato: Exploration of signal transduction pathways in cerebellar long-term depression by kinetic simulation. Journal of Neuroscience 21:5693–5702 (2001).
- F. Santamaria, S. Wils, E. De Schutter and G.J. Augustine: Anomalous diffusion in Purkinje cell dendrites caused by dendritic spines. Neuron 52: 635-648 (2006).
Kenji Doya
Introduction to reinforcement learning and Bayesian inference
The aim of this tutorial is to present the theoretical cores for modeling animal/human action and perception. In the first half of the tutorial, we will focus on "reinforcement learning", which is a theoretical framework for an adaptive agent to learn behaviors from exploratory actions and resulting reward or punishment. Reinforcement learning has played an essential role of understanding the neural circuit and neurochemical systems behind adaptive action learning, most notably the basal ganglia and the dopamine system. In the second half, we will familiarize ourselves with the framework of Bayesian inference, which is critical in understanding the process of perception from noisy, incomplete observations.
Suggested reading
- Doya K: Reinforcement learning: Computational theory and biological mechanisms. HFSP Journal, 1(1), 30-40 (2007)
Free on-line access:
http://dx.doi.org/10.2976/1.2732246http://dx.doi.org/10.2976/1.2732246 - Doya K, Ishii S: A probability primer. In Doya K, Ishii S, Pouget A, Rao RPN eds. Bayesian Brain: Probabilistic Approaches to Neural Coding, pp. 3-13. MIT Press (2007).
Free on-line access:
http://mitpress.mit.edu/catalog/item/default.asp?ttype=2&tid=11106http://mitpress.mit.edu/catalog/item/default.asp?ttype=2&tid=11106
Boris Gutkin
Theoretical approaches to dynamics of neuronal excitability: spike generation, neuromodulation, synchrony and oscillatory dendrites
Neurons are far from passive integrators of their inputs. Ample data shows that in contrast to linear integrate and fire models, neurons perform active non-linear computations hence the structure if their activity is only partially related to the structure of the input. A classical example of such phenomenon is the voltage dependent mechanism for spike-generation explained Hodgkin and Huxley. More modern example include generation of various kinds of bursts, intermittency in spiking and intrinsic voltage-dependent non-linearities in the dendrties (dendritic spikes).
Over the path of my career, I have been interested in how the properties of neuronal excitability influence the behavior of neurons and furthermore how neuromodulators (e.g dopamine, acetylcholine) change this dynamics. By dynamics of neural excitability I mean the active spike generation, regenerative neural firing, and active dendritic properties. In my lecture I will discuss a geometric approaches to spike generating dynamics, show how to characterize these with Phase Response Functions and go over the weakly-coupled oscillator theory that allows us to link the spike-generating dynamics to collective network behavior. I will also show evidence of how neuromodulation shapes the spike-generation and what that implies to synchrony and asynchrony of networks. In the second part of the lecture I will show how the same mathematics can be used to understand the dynamics of active dendrites that generate oscillations. In conclusion I will show how we can use this theory to understand the possible mechanisms for the formation of the entorhinal grid-fields and general implications for dendritic integration.
Suggested reading
- E.M. Izhikevich: Dynamical Systems in Neuroscience The MIT Press (2007)
- Brown E, Moehlis J, Holmes P.: On the phase reduction and response dynamics of neural oscillator populations. Neural Comput. 16(4):673-715 (2004)
- Stiefel KM, Gutkin BS, Sejnowski TJ.: Cholinergic neuromodulation changes phase response curve shape and type in cortical pyramidal neurons. PLoS One. 2008;3(12):e3947 (2008)
- Ermentrout B, Pascal M, Gutkin B.: The effects of spike frequency adaptation and negative feedback on the synchronization of neural oscillators. Neural Comput. 13(6):1285-310 (2001)
- Gutkin BS, Ermentrout GB, Reyes AD: Phase-response curves give the responses of neurons to transient inputs. J Neurophysiol. 94(2):1623-35 (2005)
- London M, Häusser M.: Dendrites and grids Dendritic computation. Annu Rev Neurosci 28:503-32 (2005)
- Hafting T, Fyhn M, Molden S, Moser MB, Moser EI.: Microstructure of a spatial map in the entorhinal cortex. Nature 11;436(7052):801-6 (2005)
- Michiel W.H. Remme, Máté Lengyel, Boris S. Gutkin: Democracy-Independence Trade-Off in Oscillating Dendrites and Its Implications for Grid Cells. Neuron, Volume 66, Issue 3, 429-437 (2010)
Jeanette Hellgren Kotaleski
Microcircuits involved in motion – insights gained through modeling
One important goal of computational modeling is to synthezise data received from experiments, and predict the computational role of the different elements in a more physiological context, e.g. by scaling up the system or by extrapolating to ongoing network activity. To understand the computations going on in various neural systems, the microcircuitry is an important starting point. Understanding the microcircuit requires, however, a multi-scale approach. I will in my tutorial discuss insights gained about the microcircuitry in motor systems through recent modelling work. I will highlight the longstanding work related to the motor system in the lamprey. The lamprey is one of the few vertebrates in which the cellular and synaptic level mechanisms for goal-directed motor behaviour, including locomotion, steering and control of body orientation, are well described. Also recently the basal ganglia have been delineated in the lamprey. The insights gained from combining large-scale computational modeling with detailed experiments are reviewed. We are today for example able to model the motor system with biophysically detailed neurons and with the approximate number of neurons that are responsible in the behaving animal. I will show how activity in the whole motor network, including direction and speed of motion can be controlled from only a subpart of the network, highlighting the self-organizing nature of biological neuronal networks.
Suggested reading
- Kozlov A, Huss M, Lansner A, Kotaleski JH, Grillner S: Simple cellular and network control principles govern complex patterns of motor behavior Proc Natl Acad Sci USA, 106:20027-32 (2009)
- Hjorth J, Blackwell KT, Kotaleski JH.: Gap junctions between striatal fast-spiking interneurons regulate spiking activity and synchronization as a function of cortical activity J Neurosci. 29:5276-86 (2009)
- Djurfeldt M, Hjorth J, Eppler JM, Dudani N, Helias M, Potjans TC, Bhalla US, Diesmann M, Kotaleski JH, Ekeberg O.: Run-time interoperability between neuronal network simulators based on the MUSIC framework Neuroinformatics 8:43-60 (2010)
Eugene Izhikevich
Large-scale modeling of the brain
I will describe all the major steps in building a detailed large-scale thalamocortical model based on experimental measures in several mammalian species. The model spans three anatomical scales. (i) It is based on global (white-matter) thalamocortical anatomy obtained by means of diffusion tensor imaging (DTI) of a human brain. (ii) It includes multiple thalamic nuclei and six-layered cortical microcircuitry based on in vitro labeling and three-dimensional reconstruction of single neurons of cat visual cortex. (iii) It has 22 basic types of neurons with appropriate laminar distribution of their branching dendritic trees. The model simulates one million multicompartmental spiking neurons calibrated to reproduce known types of responses recorded in vitro in rats. It has almost half a billion synapses with appropriate receptor kinetics, short-term plasticity, and long-term dendritic spike-timing-dependent synaptic plasticity (dendritic STDP). The model exhibits behavioral regimes of normal brain activity that were not explicitly built-in but emerged spontaneously as the result of interactions among anatomical and dynamic processes. I will describe spontaneous activity, sensitivity to changes in individual neurons, emergence of waves and rhythms, and functional connectivity on different scales.
Suggested reading
- E.M. Izhikevich: Dynamical Systems in Neuroscience The MIT Press (2007)
All relevant chapters are available online at
http://izhikevich.org/publications/dsn/index.htmhttp://izhikevich.org/publications/dsn/index.htm -
The 2008 PNAS paper (with Dr. Edelman):
http://izhikevich.org/publications/large-scale_model_of_human_brain.htmhttp://izhikevich.org/publications/large-scale_model_of_human_brain.htm
Alla Karpova
Technologies for in vivo circuit research
Understanding information processing by populations of neurons requires monitoring their activity during behavior as well as the ability to perturb this activity. Recent technical advances have given experimental neuroscientists a remarkable set of methods: extracellular electrophysiological measurement of hundrends and soon thousands of neurons simulaneously; recording of the subthreshold activity of multiple neurons in awake moving animals; visualization of somatic calcium transients in a cell-type specific manner using two-photon microscopy as well as perturbation of circuit activity using genetically-encoded tools, to name a few. Although I will only have time to speak about a subset of these methods, I will contrast the currently available tools in relation to how they are able to meet different experimental challenges as well as how they will help advance our understanding of neural circuit function from the computational point of view. Specifically, I will outline the advantages and disadvantages of current methods to measure neuronal activity in fixed and freely moving animals, and highlight how the field is attempting to combine the best of electrophysiology and imaging with freely moving behavior. In addition, I will describe current strategies for spatially and temporally precise circuit perturbations. Finally, I will discuss how these methods can be combined with identification of cell types and sub-circuits.
Suggested reading
- Dombeck DA, Khabbaz AN, Collman F, Adelman TL, Tank DW.: Imaging large-scale neural activity with cellular resolution in awake, mobile mice. Neuron 56:43-57 (2007)
- Sawinski J, Wallace DJ, Greenberg DS, Grossmann S, Denk W, Kerr JN.: Visually evoked activity in cortical cells imaged in freely moving animals. PNAS 106:19557-62 (2009)
- Seelig, J.D., Chiappe, M.E., Lott, G.K., Dutta, A., Osborne, J.E., Reiser, M.B., Jayaraman, V. : Two-photon calcium imaging from motion-sensitive neurons in head-fixed Drosophila during optomotor walking behavior. Nat. Methods (in press)
- Cardin JA, Carlen M, Meletis K, Knoblich U, Zhang F, Deisseroth K, Tsai LH, Moore CI.: Driving fast-spiking cells induces gamma rhythm and controls sensory responses. Nature 459:663-7 (2009)
- Chow BY, Han X, Dobry AS, Qian X, Chuong AS, Li M, Henninger MA, Belfort GM, Lin Y, Monahan PE, Boyden ES.: High-performance genetically targetable optical neural silencing by light-driven proton pumps. Nature 463:98-102 (2010)
Mitsuo Kawato
Cerebellar internal model, Purkinje cell LTD as supervised learning rule supported by bioinformatics, and control of learning degree-of-freedom by electrical coupling in Inferior Olive nucleus
In my first talk I will introduce one of the most influential computational models of the cerebellum: cerebellar internal theory [1]. Each microzone of the cerebellar cortex acquires an internal model of some dynamical processes outside the cerebellum while guided by climbing fiber inputs as an error signal in supervised manner. Several experimental supports to this theory are also provided. Then I will move on to a systems biology model of Purkinje cell long-term depression LTD. Spike timing dependent plasticity of LTD is understood by IP3 dynamics as the eligibility trace. Memory itself is modeled by MAP kinase positive feedback loop model and this part is well supported by a series of experiments [2,3]. In my second talk, I will discuss about functions of electrical junctions between inferior olive neurons, whose density is highest in the mammalian brain. Synchronization, rhythmicity and chaotic firing [4] of inferior olive neurons and Purkinje cells can control degrees of freedom in cerebellar supervised learning.
Suggested reading
- Kawato M: Internal models for motor control and trajectory planning. Current Opinion in Neurobiology, 9, 718-727 (1999)
- Tanaka K, Khiroug L, Santamaria F, Doi T, Ogasawara H, Ellis-Davies G, Kawato M, Augustine GJ: Ca2+ requirements for cerebellar long-term synaptic depression: role for a postsynaptic leaky integrator. Neuron, 54, 787-800 (2007)
- Ogasawara H, Kawato M: Bistable switches for synaptic plasticity. Science Signaling, Science Signaling, 2(56), pe7. (2009)
- Schweighofer N, Doya K, H. Fukai, Chiron JV, Furukawa T, Kawato. M: Chaos may enhance information transmission in the inferior olive. Proc Natl Acad Sci USA., 101, 4655-4660 (2004)
Thomas Knöpfel
Introduction to modeling voltage-gated channels and interpretation of optical imaging data
Voltage-gated ion channels provide the ionic mechanisms that along with passive membrane properties determine the basic electrical behavior of nerve cells. For numerical simulations (compartmental models) the time and voltage-dependent properties of voltage-gated ion channel species are generally well described by the general formalism formulated by Hodgkin and Huxley in the 50ths where transitions between opened (conducting) and closed (non-conducting) states are determined by voltage-dependent rate constants.
After introducing these basics, I will highlight potential problems that frequently arise from the discrepancy between real electrophysiological data and idealistic modeling.
In the second portion of my presentation I will introduce optical imaging methods. The focus will be on interpreting imaging data with the aim to use them as a data source for computational models of single cells and large neuronal circuits. Finally, I will talk about the emerging optogenetic approaches that appear particularly well suited as a link between cellular neurophysiology and circuit modeling.
Suggested reading
- Quadroni R, Knöpfel T: Compartmental models of type A and type B guinea pig medial vestibular neurons. J Neurophysiol. 72:1911-1924. (1994)
- Akemann W, Knöpfel T: Interaction of Kv3 potassium channels and resurgent sodium current influences the rate of spontaneous firing of Purkinje neurons. J Neurosci. 26:4602-4612.
- Knöpfel T, Díez-García J, Akemann W.: Optical probing of neuronal circuit dynamics: genetically encoded versus classical fluorescent sensors. Trends Neurosci. 29:160-166. (2006)
- Perron A, Mutoh H, Launey T, Knöpfel T: Red-shifted voltage-sensitive fluorescent proteins. Chem Biol. 16:1268-1277. (2009)
- Perron A, Mutoh H, Akemann W, Gautam SG, Dimitrov D, Iwamoto Y, Knöpfel T.: Second and third generation voltage-sensitive fluorescent proteins for monitoring membrane potential. Front Mol Neurosci. (2009) PMID: 19623246
Klaus Obermayer
Network Models of Primary Visual Cortex
Primary visual cortex in higher animals like primates and cats is one of
the best characterized cortical areas and serves as a paradigmatic area
for understanding visual processing and cortical computation in general.
In my presentations I will describe computational approaches based on network
models of different complexity (from rate models to spiking models) and
discuss, how those models can be applied in oder to evaluate hypotheses
about the response properties of visual cortical neurons. Topics will cover
the computation of feature selective responses, the modulation of neural
response properties by stimulus context, the role of background activity
and noise, neural adaptation and activity dependent plasticity, and
implications for neural coding and the representation of visual information.
Suggested reading
- Marino J., Schummers J., Lyon D., Schwabe L., Beck O., Wiesing P., Obermayer K. and Sur M.: Invariant Computations in Local Cortical Networks with Balanced Excitation and Inhibition Nat. Neurosci. 8, 194-201 (2005)
- Schwabe L. and Obermayer K.: Adaptivity of Tuning Functions in a Generic Recurrent Network Model of a Cortical Hypercolumn J. Neurosci. 25, 3323-3332. (2005)
- Stimberg M., Wimmer K., Martin R., Schwabe L., Marino J., Schummers J., Lyon D., Sur M. and Obermayer K.: The Operating Regime of Local Computations in Primary Visual Cortex Cerebral Cortex 19, 2166-2180. (2009)
- Young J., Waleszczyk W., Wang C., Calford M., Dreher B. and Obermayer K.: Cortical Reorganization Consistent with Spike Timing but not Correlation-dependent Plasticity Nat. Neurosci. 10, 887-895. (2007)
Izumi Ohzawa
Extending the notion of receptive field for studying high-order visual neurons
A standard definition of the receptive field of a visual neuron is the area of visual space in which stimuli can influence responses of the neuron. Applying this definition to neurons in high-order areas along the visual pathway is uninteresting, because receptive fields just become increasingly larger in high-order visual areas, providing minimally useful information about the stimulus specificity. I will describe our recent efforts in extending the notion of receptive fields to more useful space that is not necessarily limited to spatial dimensions. Examples of actual experimental measurements of such receptive fields are presented.
Suggested reading
- Nishimoto S, Ishida T, Ohzawa I.: Receptive field properties of neurons in the early visual cortex revealed by local spectral reverse correlation. J Neurosci. 22;26(12):3269-80 (2006). PMID: 16554477
- Tanaka H, Ohzawa I.: Surround suppression of V1 neurons mediates orientation-based representation of high-order visual features. J Neurophysiol. 101(3):1444-62 (2009). PMID: 1910945
Tatyana Sharpee
Maximally informative irregularities in neural circuits
The question of how the nervous system achieves reliable computation using unreliable components has long interested scientists going back to John von Neumann. This is now a topic of renewed interest not only in neuroscience but also in engineering. In neuroscience, the unreliability in neural circuits is thought to be tied to the remarkable energy-efficiency of neural information processing, whereas in emerging nanotechnologies a significant number of faulty components may be impossible to isolate necessitating the need for extensive error-correction at the systems level.
In the first part of this talk, I will describe a theoretical framework for finding optimal sigmoidal input/output functions for biological networks based on the concept of decision boundaries. We will compare solutions for Gaussian, and more naturalistic exponential (Laplace) stimulus distributions, for independent and interacting neurons, and examine when and how optimal solutions change as a function of noise in individual neurons.
The second part of the talk will be devoted to applications of this approach to retinal circuits. We will discuss how nearly optimal solutions can be obtained in the retina by balancing two types of irregularities: those in receptive field (RF) shapes and those of RF center positions against each other. Either the scatter in receptive field center positions or irregularities in RF shapes would have been significantly detrimental for the retinal performance alone. A comparison with data on RF mosaics in the primate retina reveals that information maximization can predict irregularities in individual RFs shapes from the knowledge of RF center positions. Thus, in the retina we find one mechanistic demonstration of how irregular neural circuits could achieve a near-optimal performance.
Suggested reading
- T.O. Sharpee, T and W. Bialek: Neural decision boundaries for maximal information transmission PLoS ONE, 2(7) e646 (2007)
- Yuan Liu, C.F. Stevens, and T.O. Sharpee: Predictable irregularities in retinal receptive fields PNAS, 38, 16499-16504 (2009)
- J. D. Fitzgerald and T. O. Sharpee: Maximally informative pairwise interactions in networks Phys. Rev. E, 80, 031914 (2009)
PDFs are available at
Nelson Spruston
Mechanisms of dendritic integration and plasticity
This lecture will summarize work, done over the course of the last decade and more, on the subjects of dendritic integration and plasticity in pyramidal cells. The focus will be on work from my own lab, but I will also describe quite a bit of work from other labs that reinforce (or in some cases challenge) ideas that have emerged from our work. The main goal will be to convey our current thinking about how pyramidal neurons function. Both in the hippocampus (where we do most of our work) and in the cortex, pyramidal neurons have two dendritic trees: a basal tree and an apical tree. Collectively, these dendrites receive input from tens of thousands of synapses. How are these inputs integrated to determine when the cell should fire an action potential? Where in the cell does the action potential begin and how does it propagate in the dendrites? What kinds of electrical events in dendrites can lead to synaptic plasticity? An important aspect of answering these difficult questions it to understand how myriad types of ion channels function in the context of a complex dendritic tree. To address this problem, my laboratory has combined experimental and computational approaches. A major focus of the lecture will be on how these two approaches can be effectively combined in a synergistic way to advance a better understanding of dendritic integration and plasticity.
Suggested reading
- Hille B: Chapter 1 of "Ion Channels of Excitable Membranes," 3rd edition. Sinauer Associates, Sunderland, Massachusetts, USA.
- Spruston N: Pyramidal neurons: dendritic structure and synaptic integration. Nature Reviews Neuroscience, 9:206-221 (2008)
- Häusser M, Spruston N, Stuart G.: Diversity and dynamics of dendritic signaling. Science, 290:739-744 (2000)
- Kampa BM, Letzkus JJ, Stuart GJ.: Dendritic mechanisms controlling spike-timing-dependent synaptic plasticity. Trends Neurosci. 30:456-463 (2007)
Optional Reading:
- Spruston N, Häusser M, Stuart G.: Dendritic integration. In: Dendrites, 2nd edition, Stuart G, Spruston N, Häusser M, eds. Oxford University Press, 2nd edition, pp. 351-399 (2008).
- Stuart G, Spruston N, Sakmann B, Häusser M.: Action potential initiation and backpropagation in neurons of the mammalian central nervous system. Trends in Neurosciences, 20:125-131 (1997)
- Lisman J, Spruston N.: Postsynaptic depolarization requirements for LTP and LTD: a critique of spike timing dependent plasticity. Nature Neuroscience, 8:839-841 (2005)
- Sjöström PJ, Rancz EA, Roth A, Häusser M.: Dendritic excitability and synaptic plasticity. Physiol Rev. 88:769-840 (2008)
Klaus Stiefel
Introduction to modeling morphologically detailed neurons
I will first motivate the study of single neurons by showing how many fascinating computation they can carry out. Then I will introduce cable-theory, the mathematical description of current flow in dendrites. From cable theory, I will then move to multi-compartmental modeling, the simulation of complex morphologically detailed models of neurons. I will show the usefulness of multi-compartmental modeling by talking about some classic and insightful studies using this technique. I will briefly discuss non-electrical ways of information processing in neurons and how they can be modeled.
Finally, I will talk about some of the research dealing with modeling complex morphologies in our lab, particularly the generation of optimized neuronal morphologies for chosen computational functions.
Suggested reading
Students
- Abiva, Jeannine
- Ahmadi, Mandana
- Arizumi, Nana
- Bush, Jeffrey
- Bölinger, Daniel
- Clemens, Jan
- Cury, Kevin
- Friedrich, Johannes
- Ganguli, Deep
- Giacomantonio, Clare
- Herreros, Ivan
- Hesse, Janina
- Jimenez Rezende, Danilo
- Kanitscheider, Ingmar
- Kubánek, Jan
- Kurikawa, Tomoki
- Lindahl, Mikael
- Luo, Ray
- Pérez Schuster, Verónica
- Romani, Armando
- Schiess, Mathieu
- Singh, Anand
- Taouali, Wahiba
- Tsai, Ming-Chi
- Williford, Jonathan
- Yamashita, Okito
- You, Hongzhi
- van Versendaal, Danielle
Jeannine Abiva
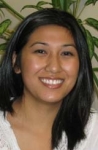
Affiliation: |
The University of Iowa |
URL: | http://myweb.uiowa.edu |
About: |
I have just started to work on a project that studies learning and memory. For this project, I will need to know how to interpret the multi-unit recording data to create a model for episodic memory. Since the University of Iowa does not offer a course in computational neuroscience, the Okinawa Computational Neuroscience Course will allow me to learn the skills of neural data analysis and modeling of neural activity, both essential to my research. |
Mandana Ahmadi
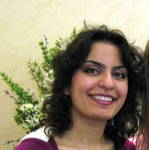
Affiliation: |
Gatsby Computational Neuroscience Unit, UCL |
URL: | http://www.gatsby.ucl.ac.uk/~mandana |
About: |
I am interested in understanding adaptive goal-directed behaviour and its implementation at a neuronal level. In this course I hope to interact with and learn from leading experts in several sub-fields of neuroscience. This opportunity provides me with a complementary training to my current graduate studies, offering me a broader view for addressing the problems I am interested in solving. I am also looking forward to rich interactions with my fellow students. |
Walther Akemann
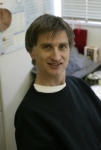
Affiliation: |
RIKEN Brain Science Institute 2-1 Hirosawa, Wako-City Saitama 351-0198, JAPAN |
URL: | http://www.brain.riken.jp/common/cv/w_akemann.pdf |
About: |
It is my pleasure to join OCNC 2010 as a tutor for a second time. This course provides a rare opportunity to advanced students and beginning researchers to explore new ideas away from the beaten track that you follow in our daily work, but yet may inspire new paths to follow in the future. The course brings together a diverse set of people with a common interest for computational biology and neuroscience. My own background is in neuroscience, physics and electrochemistry. Presently, I am a staff scientist at the RIKEN Brain Science Institute in Tokyo (Japan) and my research there is about functional dissection of cortical circuits of the mouse sensorimotor system using optogenetic probes. The brain, on many levels of its organization, performs model simulations to predict future events using internal representations of the physical world and multiple sensory feedbacks. Successful models suggest a gain of competence and understanding in dealing with the world. I am looking forward to meet you at OCNC 2010 and wish all participants a worthwhile learning experience while creating new models, trying new computational strategies and, on the way, enjoying the many emotional leaps that go along with deeper understanding. |
Shun-ichi Amari
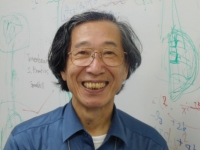
Affiliation: |
RIKEN Brain Science Institute |
URL: | http://www.brain.riken.jp/labs/mns/amari/home-E.html |
About: |
I am a mathematician interested in mathematical neuroscience as well as other mathematical sciences and engineering. I have worked on statistical neurodynamics, neural field theory, associative memory mechanisms, learning and self-organization. My recent studies are statistical and probabilistic analysis of neuronal spikes sequences, in particular, their higher-order correlations. How information is encoded in terms of spikes and what is loss of information by neglecting some quantities in the spikes sequences. I use information geometry to elucidate those questions. |
Gordon Arbuthnott
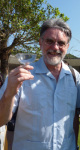
Affiliation: |
OIST |
URL: | https://www.oist.jp/bmbu |
About: |
I've been fascinated by dopamine and its action in brain since it was first described as a neurotransmitter during my Ph.D. time. The first cells we could image in brain were the catecholamine neurons and as we began to understand their anatomy it was clear that we were not dealing with the kind of system of neurons with which we were familiar from the motoneurons and sensory neurons that had been studied in depth by electrophysiologists. Now that there are computational hypotheses about what the dopamine cells signal to the organism we have a new chance to look at how they might do that and my recent work has been aimed at trying to put some flesh on the bones of that idea. I've developed some cell culture methods with which to try to understand how the connectivity in the striatum (the area that receives dopamine input) is changed by its release. The answers are not in yet but the methods are operational. |
Nana Arizumi
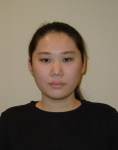
Affiliation: |
Computer Science department, University of Illinois, Urbana-Champaign USA |
About: |
As a fifth year graduate student in computer science, I am currently planning the next stage of my life. I specialize in high speed computing, but I am currently pursuing an additional interest in computational neuroscience. The field stimulates my intellectual curiosity, because there are so many interesting applications and opportunities that I further want to learn. I believe that the Okinawa Computational Neuroscience Course is the perfect opportunity to gain such knowledge and experience. |
Romain Brette
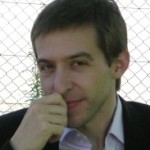
Affiliation: |
Ecole Normale Supérieure, Paris |
URL: | http://audition.ens.fr/brette/ |
About: |
My principal research theme is spike-based computation in the auditory system, in particular sound localization and pitch perception. This includes several topics: * Spiking neuron models: what is a good neuron model? I am interested in phenomenological spiking models that can reproduce the responses of real neurons in terms of spike timing. One particular aspect we are currently investigating is how the spike threshold varies with the membrane potential. We are also studying the general properties of spiking models, such as coincidence detection and reliability of spike timing. * Spike-based computation: how do neurons compute with spikes? For mathematical convenience, many approaches describe neural computation in terms of population firing rates. I am interested in the intrinsically spike-based aspects of computation, in particular related to synchrony. I am looking at the relationship between stimulus-induced synchrony and structural properties of stimuli for several computational problems in perception, such as sound localization. * Simulation of spiking neural networks: we have developed a spiking network simulator in Python called Brian (http://briansimulator.org), to make writing models simple and flexible. We are focusing now on parallel simulation, mainly using graphics cards (GPU). |
Jeffrey Bush
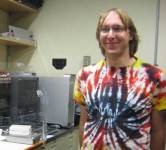
Affiliation: |
Neurosciences Graduate Program, UCSD |
About: |
I am a first year graduate student at UCSD. I have an undergraduate background in molecular biology and computer science. I intend to learn how to transition from experimental data into computational models, along with learning new experiments and analysis techniques. Additionally I wish to expand my knowledge about other areas of the brain, the computations they perform, and how they are being studied. I have wanted to travel to Japan since I was a kid when my family hosted a foreign exchange student from Japan. |
Daniel Bölinger
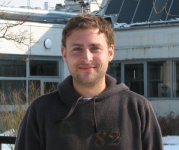
Affiliation: |
Visual Coding Group Max-Planck-Institute of Neurobiology Munich, Germany |
URL: | http://www.neuro.mpg.de/english/junior/visualcode/ |
About: |
I have been attracted to neuroscience since my studies of physics. I find it intriguing that there is such a wide range of methods and techniques that, if used wisely, might provide spectacular insights to fascinating questions. I am coming to OCNC to broaden my overview of computational methods, getting to know especially those which have not yet been part of my research or which I never knew they exist. Further knowledge in data analysis and obtaining new skills in modeling would allow me to approach my scientific questions from a new point of view. Moreover, I am looking forward to meet the faculty and a world-wide selection of students in an informal atmosphere. I hope the course will offer the opportunity for discussions and the exchange of experiences in science and beyond. Last but not least, I am very fascinated by Japan, its people and traditions. Therefore, and because it is my first visit, I eagerly anticipate the "Japanese experience". |
Jan Clemens
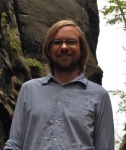
Affiliation: |
Humboldt Universität zu Berlin, Department of Biology and BCCN Berlin |
URL: | http://www2.hu-berlin.de/biologie/vhphys/clemens.html |
About: |
I think OCNC2010 is a great opportunity to meet other like-minded people who share my interest in neural coding. I have asked people who have attended the course in previous years and who found the atmosphere stimulating and vibrant. I hope that during the lectures, the project, as well as the "extra-curricular" activities I will meet nice people and maybe even make new friends. In addition, through the project and the lectures, I hope to learn a lot of new stuff. I especially want to get started with the modeling of a simple sensory network (my project). I have so far mostly done analysis of neural data and have thus little hands-on experience in modeling. And last but not least, I've never been to Japan before. |
Kevin Cury
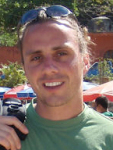
Affiliation: |
Harvard University |
URL: | http://golgi.harvard.edu/Faculty/faculty_profile.php?f=naoshige-uchida |
About: |
Basically, I would like to learn methods in computational modeling. In particular, I'm interested in creating a systems-level model of the olfactory bulb in an effort to identify the neural underpinnings behind various phenomena I have observed in my own recordings. For example, I would like to explore the interplay between excitatory and inhibitory neurons in how it impacts the distribution of activity in space and time. |
Peter Dayan

Affiliation: |
Gatsby Computational Neuroscience Unit, UCL |
URL: | http://www.gatsby.ucl.ac.uk/~dayan |
About: |
I build mathematical and computational models of neural processing, with a particular emphasis on representation and learning. The main focus is on reinforcement learning and unsupervised learning, covering the ways that animals come to choose appropriate actions in the face of rewards and punishments, and the ways and goals of the process by which they come to form neural representations of the world. The models are informed and constrained by neurobiological, psychological and ethological data. |
Erik De Schutter
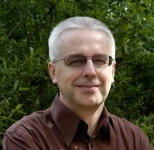
Affiliation: |
OIST |
URL: | http://www.irp.oist.jp/cns/ |
About: |
I have been teaching for more than 10 years at European CNS summer schools and was part of the last four OCNCs. It is always exciting to meet the diverse groups of highly motivated young scientists attending our courses. Summer courses have an important function in teaching computational methods and approaches, and in establishing social networks among the participants. Ideally every neuroscientist, including experimentalists and clinicians, should attend a CNS course because computational methods have become essential tools to understand the complex systems we are studying. There is a broad range of modeling approaches available. I have specialized in data-driven, bottom-up methods that are more accessible to experimentalists because they are mainly parameter driven. This includes large compartmental models of neurons with active dendrites, networks with realistic connectivity using conductance based neuron models and reaction-diffusion models of molecular interactions. I will focus on the latter during my methods presentation, but please feel free to ask me or my collaborators about our other work! Most of the work in my lab concerns the cerebellum, including its main neuron the Purkinje cell. |
Kenji Doya
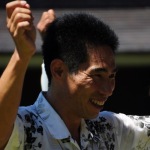
Affiliation: |
Okinawa Institute of Science and Technology |
URL: | http://www.nc.irp.oist.jp |
About: |
I have been pursuing the dual goals of creating autonomously adaptive artificial systems and understanding the flexible learning mechanisms of the brain. I am particularly interested in the functions of the basal ganglia and neuromodulatory systems in reinforcement learning and the cerebral cortex in goal-directed representation learning. |
Johannes Friedrich
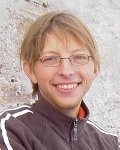
Affiliation: |
Institute of Physiology University of Bern Switzerland |
URL: | http://www.physio.unibe.ch/~friedrich/ |
About: |
I obtained my original training in Theoretical Physics and am interested in the physics of complex systems. Considering the brain as the most challenging and interesting complex system, I made a shift in direction towards neuroscience and am currently doing my PhD in Theoretical Neuroscience within the group of Walter Senn in Bern. On a wide scope my research interests are machine learning and neuroscience and what they can contribute to each other. On a smaller scope I am dealing with reinforcement learning and decision making. In particular I am interested in models for agents that face non-Markovian tasks involving delayed and uncertain reward. Since I am working on how network dynamics gives rise to a certain behavior, in my case decisions, the topics of networks and behavior offered by the OCNC fit perfectly my own research interests. Of special interest to me is the bridge between events on a neuronal scale like STDP and resulting behavior, a topic also considered by some of the lecturers. It is great to enjoy the presence world's leading experts in the area of reinforcement learning and have the chance to talk to the speakers, tutors and to each other. Besides the scientific benefit the OCNC promises, I also expect to enjoy the beautiful island, beach, ocean and social life. Looking forward to seeing you all in Okinawa! |
Deep Ganguli
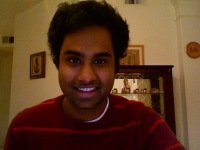
Affiliation: |
New York University |
About: |
I want to learn some new computational techniques while enjoying the scenery. |
Clare Giacomantonio
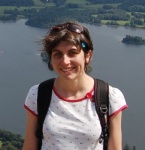
Affiliation: |
Queensland Brain Institute, The University of Queensland, Brisbane, Australia |
About: |
I am a 2nd year PhD student in Geoffrey Goodhill's lab at the Queensland Brain Institute in Brisbane, Australia. I am modelling various aspects of brain development. Previously, I have used established dimension reduction models to explore visual cortical map development. Currently, I am modelling the genetic regulation of specialised cortical areas. I work in a computational neuroscience group in an experimental neuroscience research institute, so I am looking forward to spending three weeks with neuroscientists with a more computational and mathematical focus. I hope that discussions with students, tutors and faculty, as well as exposure to new modelling methods and tools, will inspire new directions in my research. OCNC will be my first visit to Japan and I am greatly looking forward to experiencing the culture, seeing the sites and enjoying the food too! |
Cengiz Gunay
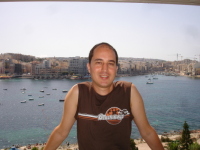
Affiliation: |
Department of Biology Emory University, Atlanta, GA 30322, USA |
URL: | http://www.biology.emory.edu/research/Prinz/Cengiz/ |
About: |
I entered computational neuroscience after an undergraduate degree in electrical engineering and a Ph.D. in computer science. I first worked as a postdoc with Dieter Jaeger on analyzing the parameter space of a multi-compartmental globus pallidus neuron based on recordings from the rat. I am currently doing my second postdoctoral fellowship with Astrid Prinz on calcium-based activity sensing mechanisms for homeostatic regulation of central pattern generating neuronal networks in lobsters. In her lab, I am also involved in building a Drosophila motoneuron model. My more general interest is in finding ways to automatically extract biologically relevant features from large numbers of simulations or experiments, organizing this information in databases, and finding efficient and novel methods for their analysis. At OCNC, I am looking forward to learn more about different computational neuroscience approaches, meeting some new people, having some fun and helping realize some cool new projects all at the same time! I made some really good friends in OCNC 2009. If you take a walk on the beach before breakfast, we would probably meet. :) |
Boris Gutkin
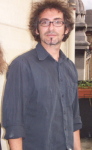
Affiliation: |
Group for Neural Theory Laboratoire de Neuroscience Cognitive INSERM U960 Departement des Etudes Cognitives Ecole Normale Superieure de Paris |
URL: | http://www.gnt.ens.fr |
About: |
The goal of my research has been to understand the dynamics and the function of neural structures: from cells to circuits to behavior. At the single cell level I strive to understand the dynamics of cell excitability, to develop a dynamical theory of action potential generation, including minimal models and to understand the role of neuromodulatory and adaptive processes in shaping these dynamics. At the circuit level I study the dynamics of collective behaviors that arise as a consequence of the cells dynamics: for example, the synchronization of neural oscillators and the sustained activity in neural network models of working memory. I am particularly interested in the role of neuromodulation in these phenomena, and further in how the biophysics of the neurons and cortical circuits may constrain the computations performed by such structures. At the behavioral level I develop models of addictive drug action and of addiction, striving to understand how biophysical mechanisms involved lead to pathological behavioral and cognitive outcomes. |
Jeanette Hellgren Kotaleski
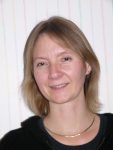
Affiliation: |
Dept Computational Biology, School of Computer Science and Communication, Royal Institute of Technology, Stockholm, Sweden |
URL: | www.csc.kth.se/~jeanette |
About: |
Jeanette Hellgren Kotaleski uses mathematical modeling to investigate the neuronal mechanisms underlying information processing, rhythm generation as well as learning in motor systems. The methodological approaches range from simulations of large-scale neural networks down to kinetic models of subcellular processes. |
Ivan Herreros
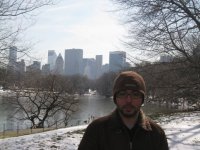
Affiliation: |
Universitat Pompeu Fabra. Barcelona. Spain. Specs-lab. |
URL: | http://specs.upf.edu/ |
About: |
Hi, I'm Ivan Herreros. I come from Barcelona. I have a degree in Computer Science from the Polytechnic University of Catalonia (UPC), in Barcelona. I did my Masters in computational linguistics in the Universitat Pompeu Fabra, in Barcelona. Afterwards I moved to Paul Verschure's lab, also in the UPF, where I started working in computational neuroscience. Since my undergraduate years I was interested in machine learning, but now what really interests me is animal learning. Currently I'm working both in modeling and data analysis of the cerebellar cortex. I try to understand and reproduce the computation performed by the cerebellum during classical conditioning of the eye-blink response. The task is tough, and I count on the OCNC to help me pursue it. In 2008 I was in a mini-summer school in Trieste and it was very helpful. I know that Okinawa will be better. That's why I'm looking forward so much to be there. Moreover, it will be my first time in Japan. See you soon. |
Janina Hesse
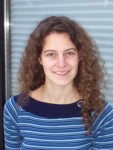
Affiliation: |
Ecole Normale Supérieure, Paris (France) |
About: |
I am totally fascinated by neuroscience and with every piece that I learn about it I am more convinced that I want to work in this area. In my opinion I need to gather as much information as possible about the brain and the different approaches to understand its complexity. Completing my master of physics in summer I am especially interested in augmenting my knowledge about biological and psychological aspects. I am sure that a course like OCNC, that spans from single neurons to behavior, will be perfectly adapted for expanding my horizon. In my research I would like to connect theory and experiment and I am looking for a PhD position in the near future. Besides that I am very curious about Asian cultures and I am happy about the opportunity to explore the Japanese culture during my visit. |
Eugene Izhikevich
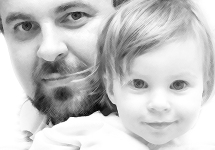
Affiliation: |
Brain Corporation, San Diego, CA |
URL: | http://www.izhikevich.org |
About: |
My research revolves around biologically detailed models of neuronal dynamics. The modeling scales span orders of magnitude, from spike-generation mechanism of individual neurons, to cortical microcircuits involving hundreds of thousand neurons, to large-scale models that have the size of the human brain (hundred billion neurons and quadrillion synapses). I strongly believe that spike timing plays an important role in brain dynamics, so I play with small-size networks (thousands of neurons) to understand the nature of neuronal computations. Recently, I started Brain Corporation to develop a spiking model of the visual system and to design specialized hardware to accelerate simulations of spiking networks. |
Danilo Jimenez Rezende
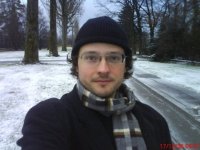
Affiliation: |
LNCO-EPFL (Laboratory of Cognitive Neuroscience) LCN-EPFL (Computational Neuroscience Laboratory (IC/SV)) |
About: |
The OCNC may be a very interesting opportunity to have feedback on my system-level modeling ideas and work. |
Ingmar Kanitscheider
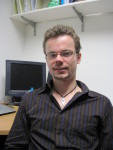
Affiliation: |
Brain & Cognitive Sciences University of Rochester |
About: |
Although my main interest lies in computational probabilistic models which link neural activity to behavior I am striving to obtain a solid and broad background in all of computational neuroscience. |
Alla Karpova
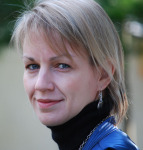
Affiliation: |
Howard Hughes Medical Institute Janelia Farm Research Center |
URL: | https://research.janelia.org/Karpova |
About: |
Dear all, I am thrilled to be at Okinawa this year. I came to systems neuroscience via a highly circuitous route. Trained as a molecular cancer biologist, I switched to neuroscience only for my postdoc when I joined Karel Svoboda's lab then at CSHL. I spent most of my time there developing molecular tools for circuit perturbation and learning about neuroscience trying to find an area that would grip my passion and would particularly benefit from the type of specific circuit manipulations that I was working on. Three years ago, I started my own lab at Janelia Farm focusing on the neural basis of decision-making in rodents. We have established a pretty broad experimental program with interesting behavioral tasks, circuit perturbations and population recordings and are just entering the modeling domain in our efforts to understand both the behavioral data as well as the neural activity. While I am excited to talk to you about many of the technological advances that make studies like ours possible, I also selfishly anticipate to learn at least as much from all of you about the computational side of things. |
Mitsuo Kawato
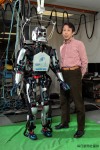
Affiliation: |
ATR Brain Information Communication Research Laboratory Group |
URL: | http://www.cns.atr.jp/~kawato/ |
About: |
Since Kenji Doya, one of my most important colleagues and OIST unit leader, moved to Okinawa, I visited this beautiful half-tropical island with exotic cultures and natures more than 10 times, and was a lecturer of OCNC three times. I love this island and am a passionate supporter of OIST. It is very rewarding to see many of pre-OCNC students very successful in neuroscience. Computational Neuroscience Neuroscience, the discipline that studies structures and functions of the brain, has developed enormously in the past 50 years. Unfortunately, its major successes are limited to elucidating brain loci responsible for some functions, and identifying substances included in some brain processes. We are still quite ignorant about information representations in the brain, as well as about information processing in the brain for specific computations. If we had enough knowledge about these, we would be able to build artificial machines or computer programs that could solve difficult problems such as visual information processing, smooth and dexterous motor control, or natural language processing. After reflecting on these past failures of conventional neuroscience research, we adopted the computational approach. That is, we construct a brain in order to understand the brain, and we understand the brain through building a brain and to the extent that we can build a brain. More concretely, we investigated the information processing of the brain with the long-term goal that machines, either computer programs or robots, could solve the same computational problems as those that the human brain solves, while using essentially the same principles. With these general approaches, we made progresses in elucidating visual information processing, optimal control principles for arm trajectory planning, internal models in the cerebellum, teaching by demonstration for robots, human interfaces based on electoromyogram, applications in rehabilitation medicine, and so on. |
Thomas Knöpfel
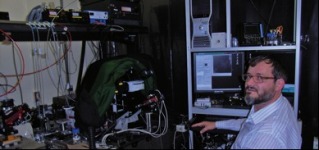
Affiliation: |
Neuronal Circuit Dynamics Brain Science Institute, RIKEN Theoretical and Experimental Neurobiology Unit |
URL: | http://neurodynamics.brain.riken.jp/ |
About: |
I was born in Germany, earned MD and Master of Science in 1985, from University of Ulm, Germany. I moved to University of Zurich, Switzerland to obtain my doctorate in physiology in 1985 and Privatdozent (PD) in 1992. In 1989 I became an assistant professor at the Brain Science Institute at the University of Zürich. In 1992, I became a team leader and project leader at Ciba-Geigy Pharmaceuticals (now Novartis). After working as a visiting professor at University College London in 1996, I joined the RIKEN Brain Science Institute as head of the Laboratory for Neuronal Circuit Dynamics in 1998. My main research interests include optical imaging with a focus on modern optogenetic methods to study the dynamics of neuronal circuits of the cerebellum and the olfactory bulb. |
Jan Kubánek
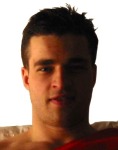
Affiliation: |
Washington University in St. Louis |
URL: | http://eye-hand.wustl.edu/ |
About: |
Computational work |
Tomoki Kurikawa
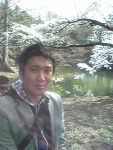
Affiliation: |
The University of Tokyo, Graduate school of Arts and Sciences. |
URL: | http://chaos.c.u-tokyo.ac.jp/index_j.html |
About: |
Hello, everyone. I'm a 2nd year Ph.D student at Univ. of Tokyo. My background is physics and dynamical systems. The master thesis is about learning model with neural networks. My ongoing study is how spontaneous activity dynamics is shaped depending on learning method and timescales between neurons and synapses. I have not received formal education about neural science, especially experimental areas, so I want to learn these topics. I'm very happy to participate in the OCNC 2010 and looking forward to meet and discuss various topics with you all. |
Yann Le Franc
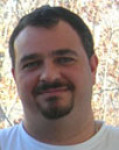
Affiliation: |
University of Antwerp |
URL: | http://www.neuroinformatics.be |
About: |
Dear all, my primary research interest is to link the impact of conductance changes (so called intrinsic plasticity) and information processing both at the level of single cell and small network. For this purpose I used both a modeling approach (using NEURON) and an experimental approach (patch-clamp, calcium imaging and dynamic clamp). More recently, the need of clear and reliable data representation and sharing to design more realistic model brought me to the Neuroinformatics field. As a member of the Task Force of the MultiScale Modeling program of the INCF I am now focused on developing an ontology of neural network models and developing a standardized language for sharing such models. I am looking forward to meeting you in OCNC and sharing with you this wonderful experience.
|
Mikael Lindahl
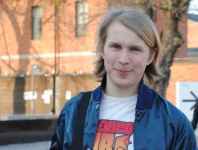
Affiliation: |
Computational Biology Royal Institute of Technology, Stockholm SWEDEN |
URL: | http://www.csc.kth.se/~lindahlm/ |
About: |
I have been pursuing my PhD for more than one year now in Jeanette Hellgren Kotaleski Computational Neuroscience lab. My passion is the basal ganglia which I use computational modeling to explore. Currently I am working on a spiking network model where I explore functional aspects on action selection of different intra nuclei connections known from experiments. During my years as an MSc student in the Sociotechnical System Engineering Program at Uppsala University I started to get fascinated by biology when learning about genetic evolution and biological dynamical systems. Now being in the field of neuroscience I feel at home and just love the challenge of trying to understand how behavior emerges from the activity of neurons. I am very much looking forward to Okinawa summer school and know it will be a fantastic experience. |
Ray Luo
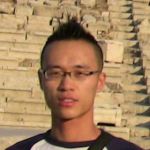
Affiliation: |
University of California, Los Angeles |
URL: | http://rayluo.bol.ucla.edu/projects/ |
About: |
I'd like to work on a project modeling granule cells in the cerebellum, and how excitatory GABARs affect signal propagation in its axons. I'd also like to learn about more general level techniques like Bayesian models in order to formulate uncertain-based probabilistic compartments. I'd like to interact with the leaders in computational neuroscience, and explore the range of scientific investigation carried out in Japan. I'd love to meet and discuss topics in brain in behavior with my computationally minded peers. |
Klaus Obermayer
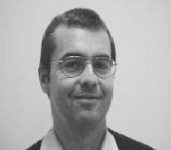
Affiliation: |
Technische Universität Berlin and Bernstein Center for Computational Neuroscience |
URL: | http://ni.cs.tu-berlin.de/ |
About: |
Our group is not only interested in understanding neural computation and sensory processing in the brain, but also in developing inductive learning methods for pattern recognition in a technical context. While the former research area belongs to computational neuroscience proper, the second research area belongs to the fields of machine learning and statistical learning, two disciplines which have been developed out of the artificial neural network community in the 80s. Why is it useful to conduct research in two fields, which do not seem to be related at first glance - except for the fact that machine learning techniques are extremely powerful tools for the analysis of neural data? Because machine learning techniques are applied in an engineering context to solve complex problems which organisms also face, the field provides a wealth of modeling tools for formulating functional hypotheses about what a piece of brain may actually compute. In my presentations I will mostly talk about techniques from computational neuroscience and about our projects on biological vision, but please feel free to ask me about this other side of our research. Over the last 20 years, computational neuroscience developed from a niche discipline, whose topics were of interest only for a small crowd of computer scientists and theoretical physicist, to a cross-disciplinary research area, whose methodologies lie at the heart of many disciplines. Concepts, models, and algorithms being developed in the computational neuroscience field, for example, became highly influencial in the design of intelligent systems in an engineering context. Also, computational modeling has begun to invade the medical sciences, for example, providing a theoretical background for understanding cognitive disabilities and neurological disorders. As a consequence, many new research centers and teaching programs have been developed in the past ten years - in Germany, for example, under the Bernstein label'' - and computational neuroscience courses have been included into the curriculum of many standard university programs, at least as an elective. |
Izumi Ohzawa
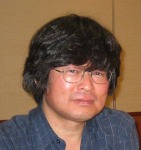
Affiliation: |
Graduate School of Frontier Biosciences, Osaka University |
URL: | http://ohzawa-lab.bpe.es.osaka-u.ac.jp/index-e.html |
About: |
My current research interests are as follows. I am primarily interested in the organization of the early to mid-stage of the visual system. How form information, including that in the 3-d space, is represented as activities of individual neurons. I am particularly interested in developing new methods for studying high-order visual neurons with minimal assumptions about what these neurons are trying to do. |
Tobias Potjans
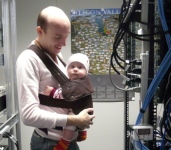
Affiliation: |
1 Computational and Systems Neuroscience, Institute of Neurosciences and Medicine, Research Center Juelich, Juelich, Germany 2 Brain and Neural Systems Team, RIKEN Computational Science Research Program, Wako, Japan |
URL: | http://www.cnpsn.brain.riken.jp/home/Tobias_Potjans |
About: |
Hi everybody, I have studied physics and computer science and I'm currently working for the Research Center Juelich in Germany and the Japanese Next-Generation Supercomputer Project. I am intrigued by the possibilities that supercomputing offers to brain science and enjoy the opportunity to scale and apply the NEST simulation tool (http://www.nest-initiative.org) to the first European peta-flop system (JUGENE, http://www.fz-juelich.de/jsc/jugene) and in future to the probably largest system in the world that is currently being built in Kobe, Japan. Nevertheless, to me it is even more important to use this massive computing power to address unresolved neuroscientific problems, such as the relation of the multi-scale connectivity structure of the brain and ongoing neuronal activity. As I have never been able to properly keep experiments and theory apart, I am very much looking forward to work with you on interdisciplinary research projects and discuss the benefits and limitations of the computational models that you want to use during the summer school and in your future research. I am very happy to come again to the fascinating island of Okinawa and hope that you find some time to learn not only about Computational Neuroscience but also about Okinawa's unique history and culture. |
Wiebke Potjans
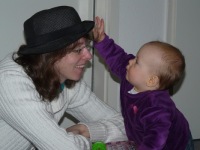
Affiliation: |
1) Computational and Systems Neuroscience, Institute of Neurosciences and Medicine, Research Center Juelich, Juelich, Germany 2) RIKEN Brain Science Institute, Wako-shi, Saitama, Japan |
URL: | http://www.cnpsn.brain.riken.jp/home/Wiebke_Potjans |
About: |
I am interested in understanding the relation between synaptic plasticity and modifications of system-level behavior. So far, I focused on spiking network models of temporal-difference learning, a variant of reinforcement learning, mostly using computer simulations. I am actively developing and using the NEST simulation tool, supplemented by various python packages in order to analyze and visualize my data. I would be happy to share my experience in reinforcement learning, especially temporal-difference learning, NEST and python with you and to learn a lot from all participants. I'm very much looking forward to come back to the tropical island of Okinawa this year! |
Verónica Pérez Schuster
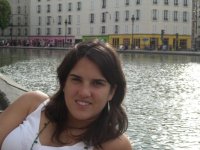
Affiliation: |
UPMC/ENS |
About: |
Hello! I come from Argentina, where I obtained my degree in Physics in the University of Buenos Aires. I did my final thesis in neuroscience, working in behavioral experiments in humans. Nowdays I'm doing my PhD in neuroscience section at the École Normale Supérieure in Paris, working with zebrafish larvae, an excellent vertebrate model for neuroscience research. I use a protocol that combines behavioral studies , two-photon calcium imaging techniques and mathematical methods for image processing and data analysis. I strongly believe that the course is a unique opportunity to develop my computational skills in a rich environment with an interdisciplinary orientation, giving me the possibility to interact and exchange ideas and experiences with people from different backgrounds. |
Armando Romani
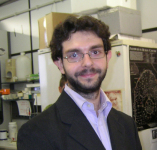
Affiliation: |
European Brain Research Institute (EBRI) |
URL: | http://www.ebri.it |
About: |
Starting in experimental research, I am now moving towards theoretical sciences. I have always been interested in mathematics, computer science and neuroscience, now I would like to combine all of these in my research. This course would be a great introduction to theoretical and computational neuroscience, and an excellent starting point to fill a missing gap in my experience. This opportunity would be even more precious for me, since I am going to collaborate with a computational neuroscience lab. I feel it is necessary for me to have a good background in this field to better understand my future work and to better interacts with my colleagues. |
Mathieu Schiess
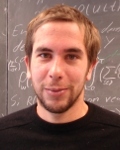
Affiliation: |
Institute of Physiology University of Bern Switzerland |
URL: | http://www.physio.unibe.ch/~schiess/ |
About: |
I am a second-year PhD student in Computational Neuroscience at the Department of Physiology of the University of Bern under the supervision of Prof. Walter Senn.I earned my Master degree in Mathematics (oriented towards dynamical systems) in 2008, following a degree in Computer Engineering (oriented to brain imaging analysis). I decided to go in for Computational Neuroscience because it is in the intersection of three of my main scientific interests : statistical physics, cognitive science and complex systems. I am working in reinforcement learning and dendritic computation. One of my main goals is to find the link between synaptic plasticity and dendritic dynamics, more precisely, how a dendritic tree could increase the computational power of a neuron to learn tasks which are not achievable by a point neuron or a network of point neurons. I am highly motivated to study and enthusiastic about learning new things. I am also looking forward to the networking opportunities generated by the diversity of people attending this summer school. |
Tatyana Sharpee
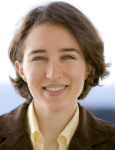
Affiliation: |
The Salk Institute for Biological Studies |
URL: | http://cnl-t.salk.edu |
About: |
The brain can perform complex object recognition tasks while consuming as little as 12 Watts of power and despite the inherent irregularities in both the responses of individual neurons and connections between them. Our group is interested in understanding the computational principles that make this possible. It has been argued that the interaction between the environment, as defined by the statistics (and dynamics) of natural sensory stimuli, and neural circuits is key for achieving such energy-efficient computation. Our recent work has been directed to examining how neurons adapt to the statistics of natural stimuli, and how coordination between irregularities of nearby neurons can yield computational power comparable to a defect-free circuit. As part of our efforts, we also develop new statistical methods for using natural stimuli to probe neural responses which we hope to use to study the high-level visual and auditory responses. |
Anand Singh
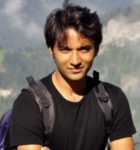
Affiliation: |
Doctoral Student University of Zurich Institute of Pharmacology & Toxicology |
URL: | http://www.pharma.uzh.ch/research/functionalimaging/members/AnandSingh.html |
About: |
I just finished the first year of my PhD in computational neuroscience. Hence I am new to the field and this summer course would be perfect at this stage to learn the fundamentals from the leaders in the field and also I will get an opportunity to interact with the fellow graduate students. The lectures on various topics will greatly enhance my knowledge about the future and challenges in the field. This experience will greatly benefit me in terms of planning and carrying out my future research more effectively. Moreover, it will pave the way for future collaborations with the researchers I will get to interact there. |
Nelson Spruston
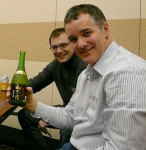
Affiliation: |
Northwestern University Department of Neurobiology & Physiology |
URL: | http://www.northwestern.edu/dendrite |
About: |
The goal of the research in my laboratory is to understand the types of integration that occur in neuronal dendrites and how the structure of the dendrites and the various types of ion channels contained in the dendritic membrane carry out the task. We study these processes using patch-clamp recordings from the soma and dendrites of neuronsmaintained in slices of living brain tissue. These recordings can be used to study the properties of both voltage-gated and synaptically activated ion channels. In addition, simultaneous recording from the soma and a dendrite of the same neuron provides information on how voltage changes such as synaptic potentials and action potentials propagate within the neuron. Data obtained from such recordings are also used in computer models incorporating the three dimensional structure of the neuronal dendritic tree. These models allow us to examine which aspects of neuronal structure and ion channel composition are critical in the process of synaptic integration, and to formulate testable predictions for future experiments. Our work also determines how these neuronal properties may change as a function of experience. Such forms of neuronal "plasticity" are widely believed to constitute the cellular basis of learning and memory. |
Klaus Stiefel
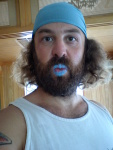
Affiliation: |
Okinawa Institute of Science and Technology Theoretical and Experimental Neurobiology Unit |
URL: | |
About: |
I got my undergraduate degree in microbiology from the University of Vienna and my PhD in zoology from the Max-Planck Institute for Brain Research in Frankfurt (with Wolf Singer). Then I did a post-doc with Terry Sejnowski at the Salk Institute in La Jolla. In 2006 I joined OIST and established the Theoretical and Experimental Neurobiology Unit. |
Wahiba Taouali
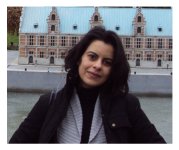
Affiliation: |
Université Henri Poincaré - LORIA, Campus Scientifique BP 239 - 54506 Vandoeuvre-ès-Nancy Cedex. France |
About: |
I apply to the OCNC because I think this course can help me focus my research onto the relevant literature as well as acquiring good modeling practices. I'm currently getting familiar with the extensive literature relative to the basal ganglia modeling as well as reinforcement learning and most of the articles I've read so far have been written by lecturers that will be present during the course. This represents for me a unique opportunity to interact with these experienced researchers and will certainly help me orienting my research in the right direction and specifying a forward plan of work. Finally, I visited China before and this will be an opportunity to be in the East again to enjoy the culture diversity. |
Benjamin Torben-Nielsen
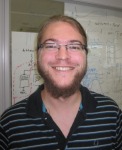
Affiliation: |
OIST |
URL: |
|
About: |
I'm interested in a lot of things. My research interests span everything in between of artificial intelligence (Alife, biorobotics) and real intelligence (cellular physiology, single-neuron computations and detailed network simulations). Currently, I'm working in the Stiefel unit at the Okinawa institute of Science and Technology (OIST) in Japan. We are working on projects that relate to the morphology and function of single neurons, and, the interaction between both. However, to study single neurons we also use large network simulations... |
Ming-Chi Tsai
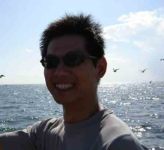
Affiliation: |
University of Alabama at Birmingham, Neuroscience program and Neurobiology department |
About: |
I am a Ph D student with Dr. Jacques Wadiche of UAB. I have my BS in Psychology from Taiwan and MSc in Neuroscience from UCL, UK, where I started to learn synaptic physiology. Currently my research involves in studying the role of glutamate transporters in cerebellar synaptic physiology. Generally, I am interested in how the neuronal circuit works to encode, transmit, and respond to the outside world by electrochemical activities and anatomical features. OCNC provides an opportunity for me to start preparing myself on this topic by means of gaining experience in computational approaches. |
Ping Wang
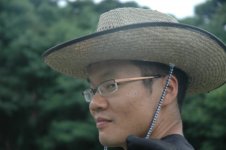
Affiliation: |
UCSD / Salk Institute |
URL: | http://cnl.salk.edu/~ping/ |
About: |
I study the role of temporal patterns in neural coding. Specifically, I am interested in quantifying the effects of synchrony on the reliability of synaptic transmission of information in thalamocortical connections. I primarily use NEURON multi-compartmental models in my studies. |
Jonathan Williford
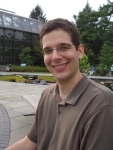
Affiliation: |
Johns Hopkins University Department of Neuroscience |
URL: | http://neuroscience.jhu.edu/ or http://neurov.is/on |
About: |
I want to gain experience in biologically plausible computational modeling, especially in relation to the visual system. My past experience in computer vision and the difficulty of many of the algorithms to achieve anywhere near the results of biological visual systems has motivated my interested in visual neuroscience. I am also excited about meeting the other participants! |
Okito Yamashita
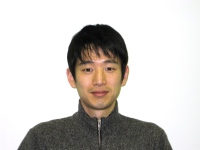
Affiliation: |
ATR Neural Information Analysis Laboratories |
URL: | http://www.cns.atr.jp/~oyamashi/index.html |
About: |
I have been working on development of data analysis methods and analysis of neuronal data (fMRI, EEG, ECoG, LFP). My approach to neuroscience research is the data-driven approach, that is, a kind of an exploratory or bottom-up way to find something from data. But it is sometimes difficult to interpret results in a more principled way. So I would like to incorporate a top-down approach into my current bottom-up approach to better understand neuroscience data. I think this course is a good opportunity to learn theoretical neuroscience. |
Hongzhi You
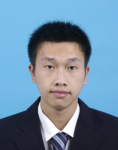
Affiliation: |
Department of Systems Science, Beijing Normal University, Beijing, China. |
About: |
In the course, I am very interested in the researchers' work about continuous attractor neuronal network and reinforcement learning of subjective value mediated by the dopaminergic neurons. Currently, two aspects of questions I concern are related to the course: 1) I attempt to model a continuous attractor neuronal network and get the analytical solutions to investigate the dynamical mechanism of multiple-choice decision making; 2) I want to establish the recurrent circuit model of spiking neurons for value-based decision making. I hope to seize this opportunity to learn and communicate with these outstanding researchers. I wish I can discuss our current work about the dynamical mechanism of multiple-choice decision making with them and share ideas with each other. |
Danielle van Versendaal
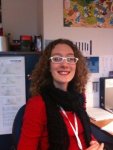
Affiliation: |
Netherlands Institute for Neuroscience |
URL: | http://www.nin.knaw.nl/research_groups/levelt_group/team/ |
About: |
My main interest is neural network development in relation to cell morphology and the balance of excitation and inhibition. Ocular dominance is a very useful model to study this. However, relating structural data to functional data is though, therefore, advancing my knowledge in computational neuroscience will be useful for interpreting my data as well as help me improve my stimulus design for calcium imaging. Furthermore, I am interested in focusing on computational neuroscience during my PhD because I suspect that modeling will give me more instant satisfaction of doing research as compared to experimental work. But in the end, I firmly believe in the powerful combination of these two approaches. |
Matthijs (Matt) van der Meer
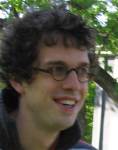
Affiliation: |
Redish lab, Department of Neuroscience, University of Minnesota |
URL: | http://www.tc.umn.edu/~vande642 |
About: |
Overall, I am interested in neural mechanisms, theoretical perspectives, and computational models relevant to planning (decision-making that takes the consequences of actions into account). I pursue these interests by recording and analyzing neural ensembles from the striatum, hippocampus, and frontal cortex in behaving rats, applying decoding, signal processing, and machine learning analyses, and building network and theoretical models of the results. |