Lecturers & Abstract / OCNC2017
Lecturers
- Upinder Bhalla (NCBS, India)
- Erik De Schutter (OIST)
- Kenji Doya (OIST)
- Bard Ermentrout (University of Pittsburgh, USA)
- Ila Fiete (University of Texas Austin, USA)
- Sonja Grün (Research Centre Jülich, Germany)
- Nicholas Hatsopoulos (University of Chicago, USA)
- Shin Ishii (Kyoto University, Japan)
- Jason Kerr (MPI Bonn, Germany)
- Bernd Kuhn (OIST)
- Sukbin Lim (NYU Shanghai, China)
- Michele Migliore (Institute of Biophysics, Italy)
- David Redish (University of Minnesota, USA)
- Greg Stephens (OIST)
- Yoko Yazaki-Sugiyama (OIST)
- Charles Wilson (University of Texas San Antonio, USA)
Abstracts
Week1. Methods (Doya(1), Yazaki-Sugiyama, Bhalla, Kuhn, De Schutter, Doya(2), and Stephens)
Week2. Neurons, Networks, and Behavior I (Ishii, Ermentrout, Wilson, Fiete, Lim, and Gruen)
Week3. Neurons, Networks, and Behavior II (Migliore, Kerr, Hatsopoulos, and Redish)
Lecturers / Brief Bio & Message to students
Upinder Bhalla (NCBS, India)
When you hear, when you move, and when you read this sentence, your brain processes events happening in sequence. We are interested in the multiscale mechanisms, from molecular to electrical to network computation, that execute such sequence computation. We have shown computationally that sequences of synaptic input arriving on short stretches of dendrite can be discriminated from disordered input. We have shown using 2-photon imaging that sequences of activity arise in the hippocampus when mice learn associations in time. We suggest that sequence-based computation may play a major part both in information processing and also in plasticity in the brain.
[Link]
Erik De Schutter (OIST)
I was born in Antwerp, Belgium, where I studied medicine and received my MD in 1984. I subsequently specialized as a neuropsychiatrist but started work on computational modeling at the same time. This led to a research career in computational neuroscience, as a postdoc at Caltech, running my own group at the University of Antwerp and since 2007 at OIST.
I taught for 19 years at European CNS summer schools and was part of the last ten OCNCs. It is always exciting to meet the diverse groups of highly motivated young scientists attending our courses. Summer courses have an important function in teaching computational methods and approaches, and in establishing social networks among the participants. Ideally every neuroscientist, including experimentalists and clinicians, should attend a CNS course because computational methods have become essential tools to understand the complex systems we are studying. There is a broad range of modeling approaches available. I have specialized in data-driven, bottom-up methods that are more accessible to experimentalists because they are mainly parameter driven. This includes large compartmental models of neurons with active dendrites, networks with realistic connectivity using conductance based neuron models and stochastic reaction-diffusion models of molecular interactions. I will not have time to present much of our research, but feel free to ask me or my collaborators about our work! Most of the work in my lab concerns the cerebellum, including its main neuron the Purkinje cell.
[Link]
Kenji Doya (OIST)
Kenji Doya took BS in 1984, MS in 1986, and Ph.D. in 1991 at U. Tokyo. He became a research associate at U. Tokyo in 1986, U. C. San Diego in 1991, and Salk Institute in 1993. He joined ATR in 1994 and became the head of Computational Neurobiology Department, ATR Computational Neuroscience Laboratories in 2003. In 2004, he was appointed as the principal investigator of Neural Computation Unit, Okinawa Institute of Science and Technology (OIST) and started Okinawa Computational Neuroscience Course (OCNC) as the chief organizer. As OIST re-established itself as a graduate university in 2011, he became a professor and the vice provost for research. He serves as the co-editor in chief of Neural Networks from 2008. He is interested in understanding the functions of basal ganglia and neuromodulators based on the theory of reinforcement learning.
[Link]
Bard Ermentrout (University of Pittsburgh, USA)
I work at the intersection of biology and mathematics. Although I pretend to be a mathematician, I have a PhD in Theoretical Biology and Biophysics from the University of Chicago. I have lectured on the material that I will present in the course for the last 25 years at a similar course in the Marine Biological Laboratory in Woods Hole, Massachusetts. I have worked on projects with students from that course and, in fact, with four of them, I am currently working on two papers. I also write software for the analysis of dynamical systems (XPPaut) which is widely used in the theoretical neuroscience community. My recent work has been on modeling the spatio-temporal dynamics in the nervous system and how it interacts with stimuli; for example how flicker can induce visual hallucinations. I am also interested in olfactory navigation: how animals find and track complex odor stimuli.
Ila Fiete (University of Texas Austin, USA)
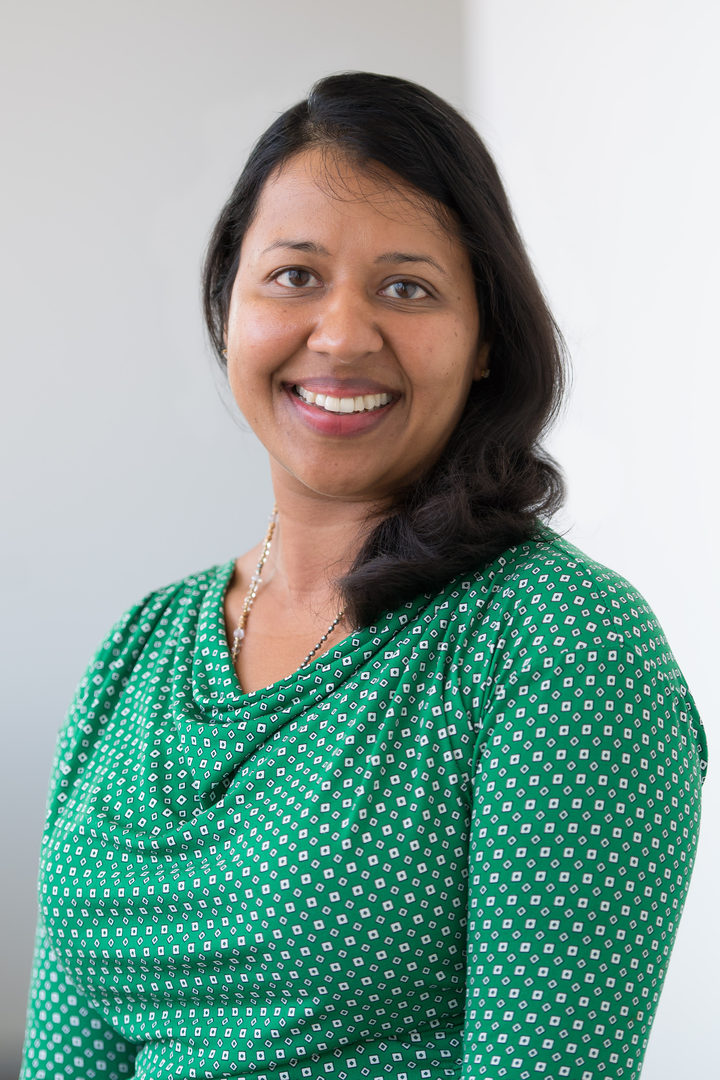
[Link]
Sonja Grün (Research Centre Jülich, Germany)
Sonja Grün, born 1960, received her Diploma in Physics from Tübingen University and a Dr. rer. nat. in Physics from Ruhr University Bochum, and her habilitation in Neurobiology and Biophysics from University of Freiburg. During her postdoc at the Hebrew University in Jerusalem she performed multiple single unit recordings in behaving monkeys. Equipped with this experience she returned back to computational neuroscience to further develop analysis tools for multi-channel recordings, first at the Max-Planck Institute for Brain Research in Frankfurt/Main, and then as Assistant Professor at Freie University in Berlin associated with the local Bernstein Center for Computational Neuroscience. From 2006 – 2011 she was Unit and later Team leader for Statistical Neuroscience at RIKEN Brain Science Institute in Wako-Shi, Japan. Since 2011 she is Vice-director of the Institute for Neuroscience and Medicine (INM-6) at Jülich Research Centre and full Professor for Theoretical Systems Neurobiology at RWTH Aachen University. She studies temporal and spatial neuronal dynamics, develops statistics for analysis of high-dimensional data, and works on data management and reproducibility.
[Link]
Nicholas Hatsopoulos (University of Chicago, USA)
One of the fundamental problems in systems neuroscience today is to understand how the activation of large populations of neurons gives rise to some of the most interesting functions of the brain, such as perception, action, learning, memory, cognition and ultimately conscious awareness. Over the past forty years, electrophysiological recordings in behaving animals have revealed considerable information about the firing patterns of single neurons in isolation, but it remains a mystery how large collections of interacting neurons mediate these functions. My overall research program is to understand how neuronal ensembles in the cortex act together to control, coordinate, and learn complex movements of the arm and hand. Using multi-electrode technology to simultaneously record from large groups of neurons, we are in a unique position to examine the activity of multiple single units in various motor cortical areas to attempt to answer two fundamental questions:
1) what motor features are encoded in single motor cortical neurons as well as in motor cortical ensembles, and
2) how these features are encoded in motor cortical ensembles.
In addition to advancing our basic understanding of the brain, this research program is contributing to a more applied research project to develop neural prosthetic systems (or brain-machine interfaces) for paralyzed patients. Our system records electrical signals from the motor cortex, decodes them into a set of behaviorally relevant output signals, and then uses these output signals to drive a computer cursor or robotic device. We are currently developing novel decoding algorithms and augmenting existing brain-machine interface systems with different forms of sensory feedback.
[Link]
Shin Ishii (Kyoto University, Japan)
Shin Ishii is now a professor of Graduate School of Informatics, Kyoto University, Kyoto, Japan, and a vice director of ATR Cognitive Mechanisms Laboratories, Kyoto, Japan. He graduated from the Univeristy of Tokyo, in 1986 (BE), and finished his master course program in 1988 (ME). He received Ph.D (U. Tokyo) in mathematical engineering in 1997. He joined Nara Institute of Science and Technology as an associate professor in 1997, where he was a full professor from 2001 to 2009.
His research topic is computational modeling of intelligence and life: systems neurobiology, statistical learning (reinforcement learning and unsupervised learning), bioinformatics and neuroinformatics.
[Link]
Jason Kerr (MPI Bonn, Germany)
I’m originally from New Zealand. I initially studied human anatomy at the Department of Anatomy and Structural Biology at Otago University in Dunedin, New Zealand. I was lucky to do my PhD in a lab in which experiments were driven by theory and modelling which meant that, as a young experimentalist, my experiments were well grounded in theoretical constructs. My doctorate was entitled “role of dopamine receptor activation in corticostriatal LTP”. From there I undertook two post-docs, one studying dendritic integration of ongoing activity, at the National Institute of Mental Health in Bethesda, Maryland, USA, and the other developing in vivo population imaging techniques at the Max Planck Institute for Medical Research in Heidelberg, Germany. I continued to work as project leader until 2006 in the department headed by Bert Sakmann. From there I was entrusted to head a research group called ‘Network Imaging Group’ at the Max Planck Institute for Biological Cybernetics
in Tübingen. It was during this time that we further developed the multi-photon imaging techniques for freely moving animals, in collaboration with Winfried Denk. These approaches also lead us to develop the ability to quantify both the head and eye positions in a freely moving animal whilst imaging neuronal population activity. Currently our long-term goal is to understand how mammals use their vision to make decisions and the neurobiological mechanisms that underlie this process. I was appointed Scientific Member of the Max Planck Society and a member of the MPI for Metabolism Research, Cologne. I’m also a Director of caesar research center, Bonn, Germany, and I head the Department of Behavior and Brain Organization.
[Link]
Bernd Kuhn (OIST)
I studied physics at the University of Ulm, Germany. For my diploma and PhD I moved to the Max Planck Institute of Biochemistry, Martinsried, Germany, focusing on the development of novel voltage-sensitive dyes and their application in cultured neurons. To optimize voltage imaging and to use voltage-sensitive dyes with two-photon excitation I accepted a postdoctoral fellowship at the Max Planck Institute for Medical Research, Heidelberg, Germany. In my second postdoc position at Princeton University, NJ, USA, I made viral vectors delivering the gene of calcium indicators and used them for in vivo imaging in the cerebellum. Additionally, I used voltage-sensitive dyes for in vivo two-photon imaging in barrel cortex of the mouse. Since 2010 I work at OIST (Optical Neuroimaging Unit). We mainly work on in vivo voltage and calcium two-photon imaging and methods development for neuroscience.
[Link]
Sukbin Lim (NYU Shanghai, China)
[Link]
Michele Migliore (Institute of Biophysics, Italy)
D.Phil. in Physics (1980, Summa cum Laude, University of Palermo, Italy). Director of the Palermo Section of the Institute of Biophysics (National Research Council, Italy), Visiting Professor of Cybernetics at the Department of Mathematics and Informatics of the University of Palermo (Italy), Visiting Professor of Computational Neuroscience at the Department of Neurobiology of the Rome "La Sapienza" University (Italy), and Visiting Scientist at the Department of Neuroscience of the Yale University School of Medicine (New Haven, USA). My lab is involved in modelling realistic neurons and networks, synaptic integration processes, and plasticity mechanisms. The main long-term goal is to understand the emergence of higher brain functions and dysfunctions from cellular processes, implementing new tools and using state of the art simulation environments on different supercomputer systems.
I have always enjoyed interaction with students, and I am sure that the OCNC will be an exciting opportunity to meet and train the next generation leaders in computational neuroscience.
[Link]
David Redish (University of Minnesota, USA)
Dr. David Redish is a Distinguished McKnight Professor of neuroscience and the J. B. Johnston Land Grant Chair in neuroscience at the University of Minnesota. He was trained in computational, theoretical, and experimental neuroscience and has contributed to our understanding of decision-making and cognition. Dr. Redish received a dual-degree BA in the writing seminars (poetry, plays) and computer science from The Johns Hopkins University, and his PhD in computer science from Carnegie Mellon University. He did postdoctoral work in neuroscience at the University of Arizona in Tucson. Dr. Redish’s research seeks to understand how our different learning, memory, and decision-making systems interact to produce behavior. Most recently, Dr. Redish has been a leader in the new movement taking an engineer’s view on psychiatric and psychological dysfunction (computational psychiatry), in which understanding information-processing dysfunctions in systems producing behavior can redefine psychiatric disorders such as addiction, PTSD, OCD, and Anorexia.
[Link]
Greg Stephens (OIST)
My research in theoretical biophysics is focused on the interface of physics and biology, broadly defined, and I explore a diverse set of problems ranging from the behavior of small organisms to the natural dynamics of human cognition. In all of my efforts I combine cross-disciplinary experimental collaborations with ideas drawn broadly from such areas as statistical physics, dynamical systems and information theory to seek unifying principles and simple, functional understanding in living systems. Trained in quantum gravity and early universe cosmology, as I switched focus, I was fortunate to experience a course such as this one and I hope that you will enjoy a similarly remarkable experience.
[Link]
Yoko Yazaki-Sugiyama (OIST)
My research interest is to understand the brain function which allows animal to behave properly. Our research unit currently working on the neuronal mechanism of the developmental ‘critical period’ where the neurons are plastic and actively shaping their network by using bird song learning. Why do birds learn to sing only in the limited time window ? We are exploring the mystery of birds’ brain to answer the questions.
[Link]
Charles Wilson (University of Texas San Antonio, USA)
I study neurons and networks in the basal ganglia. My goal is to understand why these networks contain various kinds of neurons, each with its own morphology, electrical properties, and synaptic connections within the circuit. My laboratory has characterized the ionic mechanisms of cellular dynamics in most of the well known cell types in the striatum, globus pallidus, subthalamic nucleus and substantia nigra, and in cortical neurons that innervate those structures. We have also studied the unique morphological features that characterize each cell type, and their synaptic connections of each with the other basal ganglia cells.
In my presentation for the course, I will focus on experimental application of ideas from dynamical systems. This will include examples of autonomous oscillations in single neurons, how they arise and how to dissect the mechanisms of oscillation. Neuronal resonance and entrainment of neurons by oscillations in their synaptic inputs will also be addressed using examples from the basal ganglia circuit. Some features of network properties arising from the interconnections among basal ganglia cells will also be addressed.
Dr. Wilson is Ewing Halsell Professor of Biology at the University of Texas at San Antonio.
[Link]
Go back to Top
Lecture Abstracts
Monday, June 26
Kenji Doya
Title: Introduction to numerical methods for ordinary and partial differential equations
Yoko Yazaki-Sugiyama
Title: Neuronal basis for information processing.
Abstract:
Tuesday, June 27
Upinder Bhalla
Title: Multiscale computation in the brain.
Part I: Computing with noisy chemistry. Part II: Plasticity and sequences.
Abstract:
Brain computation is highly multiscale, and emerges from a seamless interplay between many levels of physical and chemical processes. These include the familiar electrical signaling, but also reaction-diffusion processes, mechanics, and networks of genes as much as networks of neurons. To a crude approximation, electrical computation is fast, long-range, and short-term,whereas chemical computation is usually slower, local, and can lead to sustained changes.
I will introduce chemical signaling and the kinds of computation that it supports. I'll discuss the kinds of models that represent chemical signaling in different contexts, and introduce how these models are built and how they interface with electrical signaling. I'll then apply these insights to two specific multiscale computations: plasticity and sequence discrimination.
Suggested Readings:
Bhalla US.
Elife. 2017 Apr 19;6. pii: e25827. doi: 10.7554/eLife.25827.
PMID: 28422010
Molecular computation in neurons: a modeling perspective.
Bhalla US.
Curr Opin Neurobiol. 2014 Apr;25:31-7. doi: 10.1016/j.conb.2013.11.006. Epub 2013 Dec 12. Review.
PMID: 24709598
Multiscale modeling and synaptic plasticity.
Bhalla US.
Prog Mol Biol Transl Sci. 2014;123:351-86. doi: 10.1016/B978-0-12-397897-4.00012-7. Review.
PMID: 24560151
Wednesday, June 28
Bernd Kuhn
1. Ion channel physiology and the Hodgkin-Huxley model of neuronal activity
In my first lecture I will talk about electric activity in neurons. I will start with the basics of ion channels, and specifically focus on voltage-gated channels and their dynamics in response to membrane voltage. Neurons use a combination of different voltage-gated channels to generate fast (about 1 ms), depolarizing action potentials. I will explain the first action potential model by Hodgkin and Huxley . Finally, I will discuss more recent additions or fine-tuning of the time-honored Hodgin-Huxley model.
2. Functional optical imaging
Functional optical imaging has becomes one of the key techniques in neuroscience. In my second lecture I will introduce fluorescence and the most important imaging methods. I will explain what we can learn from them but also discuss their limitations.
Suggested Readings:
Thursday, June 29
Erik De Schutter
Title: Introduction to modeling neurons and networks
Abstract:
In the first talk I will discuss methods to model morphologically detailed neurons. I will briefly introduce cable-theory, the mathematical description of current flow in dendrites. By discretizing the cable equation we come to compartmental modeling, the standard method to simulate morphologically detailed models of neurons. I will discuss the challenges in fitting compartmental models to experimental data with an emphasis on active properties. The talk will finish with a brief overview of dendritic properties predicted by cable theory and experimental data confirming these predictions.
The second talk will briefly introduce network modeling. I will introduce simpler neuron models like integrate-and-fire neurons and then move on to modeling synaptic currents. I will wrap up with an overview of network connectivity.
Friday, June 30
Kenji Doya
Title: Introduction to reinforcement learning and Bayesian inference
Abstract:
The aim of this tutorial is to present the theoretical cores for modeling animal/human action and perception. In the first half of the tutorial, we will focus on "reinforcement learning", which is a theoretical framework for an adaptive agent to learn behaviors from exploratory actions and resulting reward or punishment. Reinforcement learning has played an essential role of understanding the neural circuit and neurochemical systems behind adaptive action learning, most notably the basal ganglia and the dopamine system. In the second half, we will familiarize ourselves with the framework of Bayesian inference, which is critical in understanding the process of perception from noisy, incomplete observations.
Suggested Readings:
Saturday, July 01
Greg Stephens
Title: An introduction to dynamical systems: from neural activity to natural behavior
Abstract:
My lecture will consist of two parts: an introduction to dynamical systems focused in particular on the power of qualitative analysis and a novel, quantitative approach to understanding the motion of C. elegans.
Indeed, while there has been an explosion in our ability to characterize the dynamics of molecules, cells, and circuits, our understanding of behavior on the organism-scale is remarkably less advanced. Here, we use high-resolution video microscopy to show that the space of shapes is low-dimensional, with just four dimensions accounting for 95% of the shape variance. Projections of worm shape along these four “eigenworms” provide a precise yet substantially complete description of locomotory behavior, capturing both classical motion such as forward crawling, reversals, and Ω-turns and novel behaviors such as “pause” states at particular postures. We use the eigenworms to construct a stochastic model of the body wave dynamics that predicts transitions between attractors corresponding to abrupt reversals in crawling direction and we show that the noise amplitude decreases systematically with increasing time away from food, resulting in longer bouts of forward crawling and suggesting that worms use noise to adaptive benefit.
Monday, July 03
Shin Ishii
Title: Decoding neural decision making
Abstract:
Recent advancement of various measurement technologies has allowed us to observe the central nervous system (CNS) with high temporal and special resolution. This makes it possible for artificial systems to mimic the input to or output from the CNS; such techniques are overall called ‘decoding’. In this talk, I introduce several decoding studies recently done in our group, with a particular interest in decision making performed within hierarchy of neural systems. The first is cellular decision making. Even single cells should determine their moving directions during cellular migration, employing molecular systems including Rho family GTPases. The second is neurite’s decision making; during developmental stage, axons should determine their elongation directions to perform neural wiring, which is driven by Ca2+ signals in axonal growth cones. The third is C. elegans thermotaxis; in thermal environments, worms migrate toward preferred temperature, which is driven by a CNS circuit consisting of sensory, inter, and motor neurons. The fourth is human visual attention, decoded from outside of the brain, based on EEG (electroencephalography) or fMRI. In particular, we attempt to detect neural bases that are common or different over subjects. We found resting-state activities and structure information are useful for calibrating subject-dependency in the decoder, suggesting such information is closely involved in personality.
Tuesday, July 04
Bard Ermentrout
Title: Mechanisms of excitability in simple neuronal models
Abstract:
Conductance based models will be introduced. Then I will describe hoe to analyze these using techniques from nonlinear dynamics. I will introduce the phase plane, the notion of fixed points, and bifurcations. I will classify the firing patterns of neurons based on the bifurcation behavior. Then I will consider the dynamics of neural oscillators and if time permits introduce the theory of phase models and the reduction to firing rate models in heterogeneous networks of oscillators.
Suggeted Reading & Link:
Wednesday, July 05
Charles Wilson
Title: Neuronal Oscillation and Entrainment
Abstract:
Experimental applications of nonlinear dynamics will be presented using examples from the basal ganglia. Identification of bifurcations, stability and periodicity arising from specific combinations of ion channels will be demonstrated within the limitations set by experimentally observable measures. Experimental methods for measuring phase resetting will be discussed, and phase resetting curves used to predict the variability of spike timing and the responses of neurons to transient and periodic inputs. The predictions of the phase model will be tested against experimental observations in the same neurons.
Thursday, July 06
Ila Fiete
Title: Theory of the neural circuits for spatial navigation
Abstract:
Spatial navigation involves the integration of motion cues to estimate ongoing changes in location, and the combination input from external sensory landmarks that can provide corrective cues as motion-based location estimates drift over time. Integration of motion requires that the brain possesses an analog memory that can hold information over minutes. Sensory noise and ambiguous spatial cues make self-localization during navigation computationally challenging. I will discuss strategies, in terms of dynamical computations by local mesoscale neural circuits and circuit interactions, that the brain uses to enable accurate navigation.
Friday, July 07
Sukbin Lim
Title: Recurrent network models of working memory
Abstract:
Working memory refers to an ability to hold information in mind on a time scale of a few to few tens of seconds. It is a critical component of cognitive processing such as learning, planning and decision making. Persistent neural activity in the absence of a stimulus has been suggested as a neural correlate of working memory, and theoretical works have suggested that it is maintained by recurrent network interaction that opposes intrinsic leakage of memory cells. This lecture will cover a broad range of recurrent network models for different types of working memory as well as recent advances in the experiments that may elucidate mechanisms. The first part of the lecture will cover necessary mathematical tools including analysis of spiking networks and simple asymptotic analysis.
Suggested Readings:
- Synaptic reverberation underlying mnemonic persistent activity
Wang XJ. Trends in neurosciences. 2001 Aug 1;24(8):455-63.
- Working models of working memory
Barak O, Tsodyks M. Current opinion in neurobiology. 2014 Apr 30;25:20-4.
- Balanced cortical microcircuitry for maintaining information in working memory
Lim S, Goldman MS. Nature Neuroscience. 2013 Aug 18: 16: 1306-14.
Saturday, July 08
Sonja Grün
Title: Analysis and interpretation of massively parallel neuronal data
Abstract:
Fine temporal correlations between simultaneously recorded neurons have been interpreted as signatures of cell assemblies, i.e. groups of neurons that form processing units. Evidence was found on the level of pairwise correlations in simultaneous recordings of few neurons. Increasing the number of simultaneously recorded neurons rises the expectation that cell assembly expressions are more likely to be detected due to increased sample sizes. Recent technological advances enable to record from 100 or more neurons in parallel. However, this also requires new statistical tools to perform correlation analysis on such massively parallel spike train (MPST) data which do not drown in combinatorial explosion and massive multiple testing. First approaches were based on population or pairwise measures of synchronization, and later led to methods for the detection of various degrees of higher-order synchronization and of spatio-temporal patterns. The latest techniques combine data mining with analysis of statistical significance. The lecture will give a comparative overview of these methods, their assumptions and the types of correlations they can detect.
Suggested Readings:
Monday, July 10
Michele Migliore
Title: Biophysical modeling of neurons and networks using the NEURON simulation environment
Abstract:
Understanding the neural basis of brain functions and dysfunctions has a huge impact on a number of scientific, technical, and social fields. Experimental findings have given and continue to give important clues at different levels, from subcellular biochemical pathways to behaviors. However, most of the multi-level mechanisms underlying the cognitive architecture of the involved brain regions are still largely unknown or poorly understood. This mainly depends on the practical impossibility to obtain detailed simultaneous in vivo recordings from an appropriate set of cells, making it nearly impossible to decipher and understand the emergent properties and behavior of large neuronal networks. We are addressing this problem using large-scale computational models of biologically inspired cognitive architectures. In this talk, I will present and discuss the main results and techniques used in my lab to design and exploit realistic models of neurons and networks implemented following their natural 3D structure, using the NEURON simulation environment and the olfactory bulb as an example. The main goal is to uncover the mechanisms underlying higher brain functions, helping the development of innovative therapies to treat brain diseases. Through movies and interactive simulations, I will show how and why the dynamical interaction among neurons can predict new results and account for a variety of puzzling experimental findings.
Suggested Readings:
Tuesday, July 11
Jason Kerr
Title: Easy to collect, hard to interpret: Making sense of neurosphysiology data collected in vivo using electrical and imaging techniques
Abstract:
Multiphoton-imaging allows unambiguous access to neuronal populations and neuronal substructures located well below the cortical surface. In combination with genetically encoded activity indicators this approach can be used to infer spiking activity from neuronal populations in the awake animal, with single cell and single action-potential accuracy. I will present recent imaging and analysis tools that are necessary to accurately record activity using genetically encoded calcium indicators in neuronal populations using the multi-photon excitation principle. I will also cover the basic principles of multiphoton imaging and will also outline strategies that have allowed access to neuronal activity in the freely moving animal using multiphoton excitation and recent advances in imaging techniques.
Wednesday, July 12
Nicholas Hatsopoulos
Title: Encoding of movement in the motor system
Abstract:
This lecture will describe some of the major nodes of the motor system involved in voluntary limb movement focusing mainly on the cortex but also including the cerebellum, basal ganglia, red nucleus, and spinal cord. I will discuss a major challenge in understanding what aspects of movement are encoded, if any, in these different nodes and provide some possible solutions to this challenge. I will also discuss recent developments in understanding how large neuronal populations in cortex work to plan, initiate, and execute limb movements.
Suggeted Readings:
Thursday, July 13
David Redish
Title: Computational models of decision-making systems: implications for computational psychiatry
Abstract:
We are not unitary decision-makers. Instead, our decisions arise from an interaction of multiple, competing algorithms. Looking at decision-making from an information processing perspective leads to the conclusion that there are multiple, competing algorithms evolved to select actions in different contexts. Each of these processes are instantiated by different neural circuits, and each has different vulnerabilities. I will present the neuroscience and the psychology of these different systems and their vulnerabilities. I will then discuss how an understanding of these processes can lead to novel definitions, novel treatments, and novel improvements in treatments for psychiatric disorders.
Suggested Readings:
- A. D. Redish (2016) “Vicarious Trial and Error” Nature Reviews Neuroscience 17:147-159.
For students wanting to go more in depth:
- A.D. Redish (2013) The Mind within the Brain: How we make decisions and how those decisions go wrong, Oxford University Press
- A.D. Redish and J. A. Gordon (2016) Computational Psychiatry: New Perspectives on Mental Illness. MIT Press.
All of our papers, including all of the experimental and computational modeling papers, are available from http://redishlab.neuroscience.umn.edu/Papers.html
Go back to Top