[Seminar] "Generalization in Deep Networks" by Dr. Andrzej Banburski, MIT
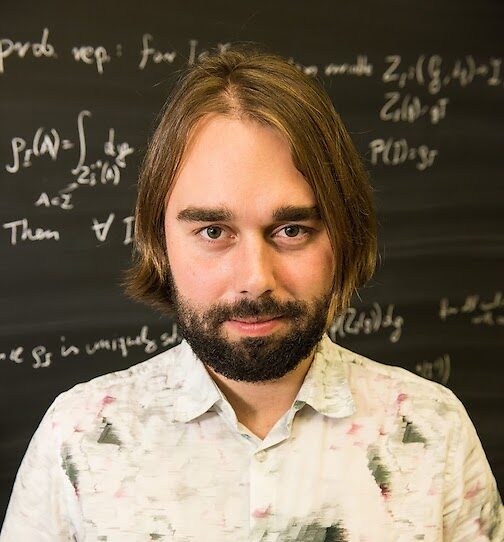
Date
Location
Description
Speaker
Andrzej Banburski. Ph.D.
Postdoctoral Researcher
Massachusetts Institute of Technology
Abstract
A main puzzle of deep neural networks (DNNs) revolves around the apparent absence of “over-fitting”, defined as follows: the expected error does not get worse when increasing the number of neurons or of iterations of gradient descent. This is surprising because of the large capacity demonstrated by DNNs to fit randomly labeled data and the absence of explicit regularization. Recent results in arXiv:1710.10345 provide a satisfying solution of the puzzle for linear networks used in binary classification.They prove that minimization of loss functions such as the logistic, the cross-entropy and the exp-loss yields asymptotic, “slow” convergence to the maximum margin solution for linearly separable datasets, independently of the initial conditions. I will show that the same result applies to nonlinear multi-layer DNNs near zero minima of the empirical loss. Interestingly, the extension to deep networks does not hold for the square loss. I will discuss natural follow-up questions from these results about which properties of different zero minima of the empirical loss characterize their generalization performance.
Subscribe to the OIST Calendar: Right-click to download, then open in your calendar application.