[Seminar] Improving Reinforcement Learning with Human Input by Professor Matthew E. Taylor
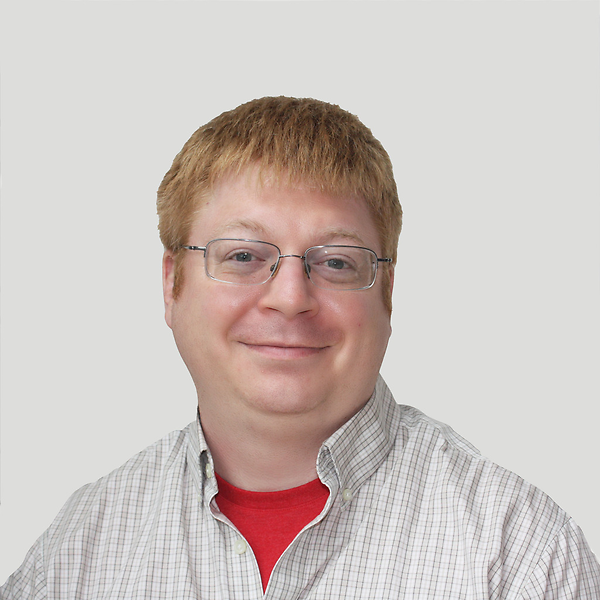
Date
Location
Description
Abstract:
Reinforcement learning has had many successes, but significant amounts of time and/or data can be required to reach acceptable performance. If agents or robots are to be deployed in real-world environments, it is critical that our algorithms take advantage of existing human knowledge. This talk will discuss a selection of recent work that improves reinforcement learning by leveraging 1) demonstrations and 2) reward feedback from imperfect users, with an emphasis on how interactive machine learning can be extended to best leverage the unique abilities of both computers and humans.
Bio:
Matthew E. Taylor received his doctorate from the Department of Computer Sciences at UT-Austin in 2008. Matt then completed a two-year postdoc at the University of Southern California and was an assistant professor at Lafayette College. He holds the Allred Distinguished Professorship in Artificial Intelligence at Washington State University in the School of EECS and is a recipient of the National Science Foundation CAREER award. Matt is currently on leave at Borealis AI, a Canadian institute funded by the Royal Bank of Canada, where he leads a research team focused on reinforcement learning.
Intra-Group Category
Subscribe to the OIST Calendar: Right-click to download, then open in your calendar application.