[Seminar] "Advances in Anomaly Detection" by Prof. Thomas G. Dietterich
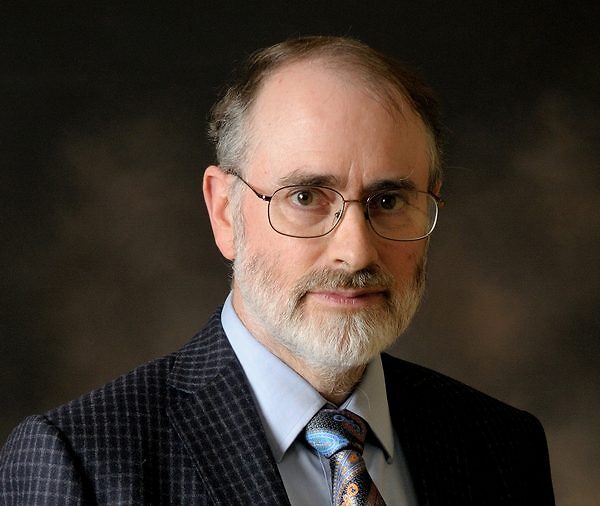
Date
Location
Description
Abstract::
Anomaly detection is important for data cleaning, cyber security, and robust AI systems. This talk will review recent work in our group on (a) benchmarking existing algorithms, (b) developing a theoretical understanding of their behavior, (c) explaining anomaly "alarms" to a data analyst, and (d) interactively re-ranking candidate anomalies in response to analyst feedback. Then the talk will describe two applications: (a) detecting and diagnosing sensor failures in weather networks and (b) open category detection in supervised learning.
Biography:
Dr. Dietterich (AB Oberlin College 1977; MS University of Illinois 1979; PhD Stanford University 1984) is Professor Emeritus in the School of Electrical Engineering and Computer Science at Oregon State University, where he joined the faculty in 1985. Dietterich is one of the pioneers of the field of Machine Learning and has authored more than 190 refereed publications and two books. His research is motivated by challenging real world problems with a special focus on robust artificial intelligence and sustainable development. He is best known for his work on ensemble methods in machine learning including the development of error-correcting output coding. Dietterich has also invented the MAXQ method for hierarchical reinforcement learning. He has a long record of service to the AI/ML community including President of AAAI, President of the IMLS, and Executive Editor of Machine Learning. He currently serves as one of the moderators for the arXiv machine learning category. He tweets as @tdietterich.
Intra-Group Category
Subscribe to the OIST Calendar: Right-click to download, then open in your calendar application.