[PhD Thesis Presentation] -Jessica Verena Schulze- Spatial and Modular Regularization in Effective Connectivity Inference from Neural Activity Data
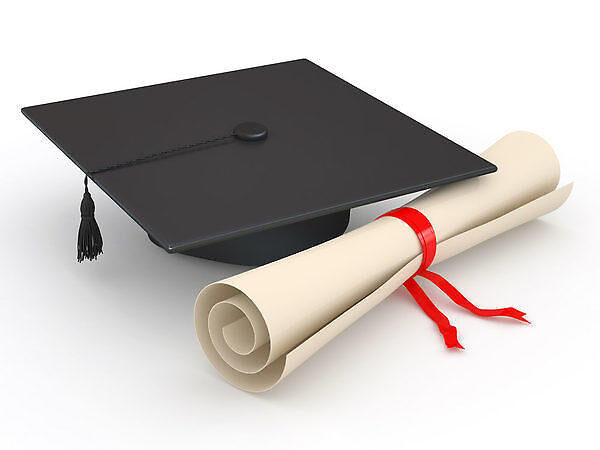
Date
Location
Description
Abstract:
Previous studies of effective connectivity inference from neural activity data applied simple regularization approaches like L1-norm regularization for sparseness.
In this thesis I investigate the incorporation of other bio-physiologically plausible priors over spatial and modular organization of neural circuits to facilitate network inference from neural activity data of large populations of neurons.
First, I incorporate distance-dependent connectivity into known techniques that impose sparseness via L1/L2 regularization in a linear non-linear poisson (LNP) cascade model. We start with simple maximum likelihood estimation with gradient decent and Newton’s method and then extend the idea of distance-based regularization to a hierarchical maximum a posteriori estimation approach with Metropolis-Hastings sampling. In this approach a prior over the existence of neural connections based on euclidian distance between neurons is included into the regularization term.
Second, I formulate a modularity prior which clusters neurons into modules and applies different model parameters for connections within each module and between modules. Then I derive a Gibbs sampling algorithm that dynamically re-assigns module membership and estimates a weight matrix.
To validate these methods, I apply them to simulated data sets with known ground truth connections weights for from up to 400 neurons. I then compare the results to previously proposed methods like model-free and simple generalized linear models (GLM) with sparse regularization.
Furthermore, I apply these methods to an extensive experimental data set of optical calcium recordings of the Posterior Parietal Cortex (PPC) and the Posteromedial Cortex (PM) of the mouse brain including populations of up to 600 neurons.
Intra-Group Category
Subscribe to the OIST Calendar: Right-click to download, then open in your calendar application.