[Seminar] Modular Deep Reinforcement Learning from Reward and Punishment for Robot Navigation
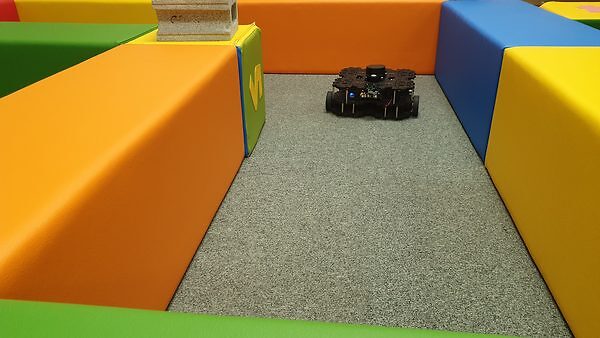
Date
2019年6月17日 (月) 15:00 〜 16:00
Location
Lab1 C015
Description
[Speaker]
Dr. Jiexin Wang
Postdoctoral Researcher, ATR
[Abdtract]
Modular reinforcement learning considers decomposing a monolithic task into several tasks with sub-goals and learning each task in parallel for solving the original problem. Some evidences recently in neuroscience show that animal utilizes separate systems for processing rewards and punishments. This forms a new perspective to modularize reinforcement learning tasks. MaxPain is one of the methods showed the advances of such dualism-based decomposing architecture over conventional Q-learning in terms of safety and learning efficiency. Deep MaxPain incorporated two convolutional neural networks to predict reward and punishment values, where the learned values were scaled into their corresponding polices. The original MaxPain combined the two values in a linear way and generated the joint policy based on the global state-action value. Deep MaxPain handled the scaling problems of numerical signals by obtaining the joint policy from the combination of two sub-policies. However, the linear weight was determined by a manually tuned parameter, resulting in an inadequate use of the learned modules. In this work, we discuss about the reward and punishment signal scaling related to the discounted factor, and propose a state-value dependent scheme to automatically determine the mixing weights. We particularly focus on the maze solving navigation tasks and investigate two metrics of pain-avoiding and goal-reaching. We show the performances in three types of mazes and illustrate the utility of different sensor fusions on Turtlebot3 Waffle Pi under gazebo simulations.
All-OIST Category:
Subscribe to the OIST Calendar: Right-click to download, then open in your calendar application.