4th Nobel Turing Challenge Initiative Workshop
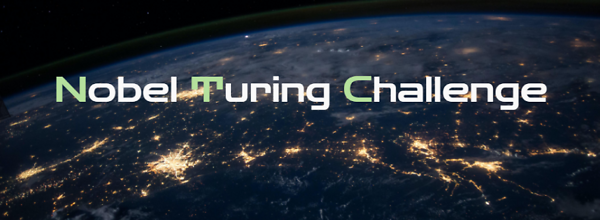
Date
Location
Description
Abstract
The Nobel Turing Challenge Initiative is a grand challenge to build a system for autonomous execution of truly meaningful scientific discovery. To accomplish this task requires objective analysis of the process of scientific discovery by humans and a deep understanding of the various technologies for autonomous execution of that process, i.e., automation of experiments and AI.
In just one year, everyone in the world can now access foundational models based on large-scale language models via the internet, and as expectations for scientific discovery are rapidly being renewed, the 4th Nobel Turing Challenge Initiative Workshop will be held in Nihombashi, Tokyo, Japan.
Topics to be discussed include, but are not limited to:
- AI for Biological Science Discovery
- AI for Materials Science Discovery
- Robotics for Scientific Discovery
- Artificial Intelligence and Human Intelligence
We hope this workshop will stimulate discussions that can only take place in early 2024, and that the momentum toward autonomous scientific discovery will become even stronger.
Your contribution will foster valuable discussions and be an asset to our workshop series.
Keynote Speakers
Shane GU, Google DeepMind, Research Scientist
Shixiang Shane Gu is a Research Scientist in Google DeepMind. Previously, he was a researcher in the ChatGPT team at OpenAI and an ex-Research Scientist at Google Research, Brain Team and a Visiting Associate Professor (Adjunct Professor) at the University of Tokyo, researching deep learning, reinforcement learning, probabilistic machine learning, and robotics. Shane holds PhD in Machine Learning from the University of Cambridge and the Max Planck Institute for Intelligent Systems, supervised by Richard E. Turner, Zoubin Ghahramani, and Bernhard Schölkopf. Shane holds B.ASc. in Engineering Science from the University of Toronto, supervised by the thesis advisor Geoffrey E. Hinton. Shane previously was also a visiting scholar at the Department of Computer Science at Stanford University hosted by Emma Brunskill. Shane's academic work received Best Paper Award at CoRL 2019 and was featured in MIT Technology Review. Shane is a Japan-born Chinese Canadian, and he speaks, reads, and writes in three languages.
Tetsuya OGATA, Waseda University, Professor
Tetsuya Ogata is a Professor with the Faculty of Science and Engineering, at Waseda University since 2012. Since 2017, he has been a Joint-appointed Fellow with the Artificial Intelligence Research Center, National Institute of Advanced Industrial Science and Technology, His current research interests include deep learning for mobile robots, manipulation, and human robot interaction.
https://ogata-lab.jp/member/ogata.html
Eran SEGAL, Weizmann Institute of Science, Professor
Eran Segal is a Professor at the Department of Computer Science and Applied Mathematics at the Weizmann Institute of Science, heading a lab with a multi-disciplinary team of computational biologists and experimental scientists in the area of Computational and Systems biology. His group has extensive experience in AI, machine learning, computational biology, and analysis of heterogeneous high-throughput genomic data. His research focuses on Microbiome, Nutrition, Genetics, and their effect on health and disease. His aim is to develop personalized medicine based on data from large-scale and deeply phenotyped human cohorts.
Prof. Segal published over 200 publications that were cited over 60,000 times, and received several awards and honors for his work, including the Overton prize, awarded annually by the International Society for Bioinformatics (ICSB) to one scientist for outstanding accomplishments in computational biology, and the Michael Bruno award. He was also elected as an EMBO member and as a member of the young Israeli academy of science. During the COVID-19 pandemic, Prof. Segal developed models for analyzing the dynamics of the pandemic and served as a senior advisor to the government of Israel.
Before joining the Weizmann Institute, Prof. Segal held an independent research position at Rockefeller University, New York.
Education: Prof. Segal was awarded a B.Sc. in Computer Science summa cum laude in 1998, from Tel-Aviv University, and a Ph.D. in Computer Science and Genetics in 2004, from Stanford University.
Lab website: http://genie.weizmann.ac.il
Program
Day One: Tuesday, February 13, 2024
10:00 - 10:15 | Welcome Note Hiroaki KITANO |
Main Keynote: AI for Science | |
10:15 - 11:00 |
Passive to Active Intelligence in Symbolic to Spatial World: From Armchair Reasoning to Experimentation Shane GU, Google DeepMind, Research Scientist Abstract: In this talk, I will discuss two trends in the evolution of artificial general intelligences. The first part focuses on learning methodologies: how scaling "passive" learning can result in a surprisingly effective "active" intelligence, capable of reasoning, tool use, and navigating through complex worlds. I will touch upon the concepts in predictive learning, online and offline reinforcement learning to highlight the promises and limitations of large-scale passive learning. The second part of the talk shifts focus to model architectures. These architectures extend beyond the realm of symbolic learning, which encompasses text, mathematics, and coding, to the rich 3D-temporal spatial world. These two trends are converging to forge an active generalist intelligence with profound understanding in both symbolic and spatial realms, spanning digital and physical worlds, with the potential to significantly enhance the capabilities of scientists in various fields. |
Session 1: AI for Biological Discovery | |
11:00 - 11:20 | Introduction to AI for Scientific Discovery in Biology Jun SEITA, RIKEN, Team Leader Abstract: In biology, the extremely complex nature of the research subject makes it quite difficult to take a science approach that determines the governing equations and performs simulations, and a data-driven approach based on observation is taken. However, the high cost of data generation makes it difficult to apply deep learning, which is significantly powerful for feature extraction. In this talk, I will give an overview of the current status of AI for scientific discovery in biology and introduce the application of reinforcement learning based on the world model to biology. |
11:20 - 11:40 | Towards an AI-based solution of protein structure prediction problem Zhang YANG, National University of Singapore, Professor Abstract: The past five years have witnessed revolutionary changes in computer-based protein structure prediction, mainly driven by the artificial intelligence (AI) and deep neural-network learning techniques. In this talk, we discuss structure prediction results in recent community-wide CASP experiments, showing that new deep-learning approaches, built on coevolution data from multiple sequence alignments, can result in consistent and successful folding of large proteins with complicated topologies. Of note, AlphaFold2 trained through end-to-end transformer networks could fold nearly all protein domains in CASP14 with 2/3 of them having accuracy comparable to low-resolution experimental solutions. In the most recent CASP15 experiment, new advancements over AlphaFold2 have been made by integrating end-to-end learning and protein language models with fragment-assembly simulations. These achievements essentially break through the 50-years-old barrier of homology-based modeling, which marked a solution, at least at the fold-level, to the single-domain protein structure prediction problem. Nevertheless, constructions of atomic-resolution models for multi-domain and higher-order protein complexes remain challenging. Given the power of AI and rapid advancement of the field, it is expected that the problems should be solved in a foreseeable future by coupling deep learning techniques and metagenome sequencing databases, with the aid of advanced structure assembly simulation algorithms. |
11:40 - 12:00 | Digitalization-driven Transformative Organic Synthesis (Digi-TOS) Takashi OHSHIMA, Kyushu University, Professor Abstract: Digitalization of organic reactions is essential for developing the next generation of organic synthesis with the assistance of artificial intelligence (AI) and machine learning (ML) methods. In this regard, we have recently launched the "Digitization-driven Transformative Organic Synthesis (Digi-TOS)," a unique digitization platform that promotes the fusion of different fields of experimental chemistry and information science for diverse organic synthesis. We are developing automated methods (molecular design, synthetic pathway search, optimization of reaction conditions, batch-to-flow conversion, and autonomous synthesis systems) that thoroughly utilize AI techniques to accelerate innovation for creating novel reactions and molecules. We will also construct our database (DB) optimized for ML in organic chemistry, which will be the basis for developing automated methods. |
12:00 - 12:20 | Language Models as Catalysts in Biomedicine: Navigating Automation, Curation, and Reproducibility in Research Sucheendra KUMAR PALANIAPPAN, The Systems Biology Institute, Senior Scientist Abstract: This talk presents our research on using Large Language Models (LLMs) in biomedical research, focusing on knowledge curation, lab automation, and reproducibility. We demonstrate how LLMs efficiently curate biomedical literature for pathway analysis and knowledge extraction. We then discuss LLMs in automating the conversion of scientific texts into machine-readable formats, enhancing lab efficiency. Lastly, we highlight the role of LLMs in improving the reproducibility of bio-related data analyses, addressing a key research challenge. This session will provide an overview of applications of LLMs in advancing biomedical research. |
12:20 - 12:40 | Using the dynamics of discovery: Generating scientific hypotheses leveraging sequences of prior insights Tarek BESOLD, Sony AI, Senior Research Scientist Abstract: Literature-based Discovery Literature-based Discovery (LBD) has regained popularity as a means to enhance the scientific research process. The resurgent interest has spurred the development of machine learning models aimed at making previously implicit connections between scientific concepts/entities explicit based on often extensive repositories of published literature. Understanding the temporally evolving interactions between these entities can provide valuable information for predicting the future development of entity relationships. However, existing methods often underutilize the latent information embedded in the temporal aspects of interaction data. |
12:40 - 13:00 | Round Table |
Session 2: AI for Material Discovery and Beyond | |
14:00 - 14:20 | RIKEN's AI for Science project: TRIP-AGIS Makoto TAIJI, RIKEN, Deputy Director Abstract: Coming soon... |
14:20 - 14:40 | Towards Automated Research in Machine Learning Wataru KUMAGAI, The University of Tokyo, Assistant Professor Abstract: The advancement of civilization has been significantly propelled by the progress in science and technology. Historically developed through human endeavor, current scientific and technological pursuits often encounter challenges inherent to human limitations. To address these challenges and enhance the efficiency of scientific development, focusing on automated research emerges as a crucial initiative. This presentation delves into the role of artificial intelligence and machine learning technologies in advancing automated research. Specifically, it focuses on how recent advancements in machine learning are instrumental in automating aspects of machine learning research itself, thereby creating a self-reinforcing cycle of innovation and discovery. |
14:40 - 15:00 | From AI and Robotics toward Generative Design of Materials Kedar HIPPALGAONKAR, Nanyang Technological University, Associate Professor Abstract: A combination of AI, high-throughput experiments (robotics) and high performance simulations can be used to accelerated materials development. I will first start by describing our efforts at lab-to-tech, where our technologies have been instrumental in successful formulation discovery, optimization as well as commercialization (both with industry partners and through our startup, Xinterra Inc.) In order to leverage these tools further, the ultimate goal is to achieve AI-driven materials discovery. Here, an important principle is that structure determines property. Therefore, property-driven generative design, driven by machine learning, critically requires understanding of the structure of materials. A deep understanding of crystal structures and their symmetries is essential for accurate invertible feature representations of materials. Next, the development of physics-aware generative models becomes critical to ensure target-oriented learning branches. Further, generated crystal structures require validation, both computationally and experimentally. After validation, the challenge is the experimental synthesis of these materials, which must be paired with data-driven characterization techniques to assess their properties. This is challenging due to the lack of a general method to rapidly synthesize and (optimally) dope bulk materials. In my work, I will discuss how all of these come together and how we address a key bottleneck by the invention of a rapid self-sintered solid-state synthesis technique (tested on GeTe, Copper, Silver Antimony Telluride), achieving phase-pure crystalline materials synthesized in the milligram scale in as little as 15 seconds. This accelerates the solid-state reaction process by a factor of >100 relative to the traditional route of mix-and-bake. |
15:00 - 15:20 | Autonomous synthesis of solid materials using machine learning and robots Taro HITOSUGI, The University of Tokyo, Professor Abstract: Global transformation is accelerating due to advancements in digital technologies, and our laboratory is evolving in tandem. In materials science research, there is a growing trend toward incorporating autonomous synthesis and characterization. This shift necessitates the integration of machine learning, robotics, and big data analytics. In this context, we present our latest achievement in autonomous materials exploration. Our system is engineered to fully automate the processes of sample handling, thin film deposition, and the refinement of growth conditions. By leveraging Bayesian optimization in conjunction with robotic automation, we facilitate high-throughput experimentation, generating extensive datasets that encompass all aspects of the deposition processes. Our experiments demonstrate the synthesis and optimization of resistance in Nb-doped TiO2 thin films, serving as a tangible example.[1, 2] This presentation concludes with a brief discussion of the prospects and profound impact of this innovative research methodology in expediting advancements in materials science. |
15:20 - 15:40 | Accelerated Material Discovery Seiji TAKEDA, IBM Research, Principal Research Scientist and Team Lead |
15:40 - 16:00 | Round Table |
16:10 - 16:20 | Link-J Host Announcement |
Session 3: Robotics for Scientific Discovery | |
16:20 - 16:40 | Robotics and AI accelerates remote automated life science Tohru NATSUME, Robotic Biology Institute Inc., Director, National Institute of Advanced Industrial Science and Technology, Prime Senior Researcher Abstract: Corona virus spread has migrated us to new normal lifestyle including remote-work. In this presentation, I will propose that remote automated life science using humanoid robots/AI. This concept would be a strong system that guarantees and validates our experiments to be innately reproducible and transferable, realizing Bio-DX (Digital Transformation) to accelerate life science research. |
16:40 - 17:00 | AI-Driven Robot Manipulators for Lab Automation Weiwei WAN, Osaka University, Associate Professor Abstract: In this talk, I will present two robotic manipulator systems that perform lab experiments with the support of AI-based recognition and inference. The first robotic manipulator system arranges test tubes into pre-defined patterns for automatic detection by conventional machines. The system autonomously collects training data for deep learning-based visual detection and leverages reinforcement learning to determine tube arrangement sequences. The second robotic manipulator system focuses on pipetting tasks. The system uses a visual transformer to detect and switch pipette tips and can perform liquid handling following human instructions. The system is integrated with the RIPPS automation system in RIKEN for chemical screening. |
17:00 - 17:45 |
Bridging the Gap: AI's Transition to Real-World Tasks Tetsuya OGATA, Waseda University, Professor Abstract: A summary of your talk, outlining the key points or focus. The current state of generative AI has seen rapid development, extending its application beyond text to various modalities such as audio and video. However, these applications primarily deal with mapping inputs to outputs. To enable AI to interact effectively in the real world, especially with physical bodies like robots, dynamic adaptive capabilities are essential. Inspired by "active inference" in neuroscience, we have been exploring "deep predictive learning" and implementing it with deep learning techniques in real robots. Our research has already enabled diverse object manipulation by robots and led to collaborations with the industry. Our moonshot project, "AIREC," aims to fuse AI and robotics to create versatile smart robots. Within this project, we plan to revolutionize how robots generalize tasks using the deep predictive learning framework. This presentation will summarize the achievements above. |
17:45 - 18:05 | Round Table |
18:05 - 18:30 | Flash Talks for the Poster Session |
18:30 - 20:30 | Poster Session and Reception |
Day Two: Wednesday, February 14, 2024
Session 4: Artificial Intelligence and Human Intelligence | |
10:00 - 10:20 | Brain-Machine Interfaces, Artificial Intelligence, and Consciousness Ryota KANAI, Araya Inc., Founder & CEO Abstract: In this talk, I will present our ongoing research and development efforts on AI-enhanced Brain-Machine Interfaces (BMI), supported by Japan's Moonshot Program. Our objective is to augment BMI capabilities by leveraging AI technologies. AIs can be used not only to improve the decoding of brain signals, but also to enhance the functionality of cybernetic avatars such as remote robots and virtual agents. Additionally, we aim to expand the scope of BMI beyond the traditional binary of invasive versus non-invasive methods, and introduce innovative approaches like minimally invasive BMIs, which record brain activities via the interior of the cortical vein. Moreover, we are advancing the development of X-Communication technologies, which enables the transmission of thoughts and concepts between brains by aligning the latent brain spaces across individuals. While these advancements target practical applications, the data and ideas generated in this project are also expected to contribute to fundamental research in neuroscience and the study of consciousness. |
10:20 - 10:40 | The worldview of manga "The Gene of AI" Kyuri YAMADA, Manga Artist, the author of “AI no Idenshi (The Gene of AI)” Abstract: The Japanese science fiction manga "The Gene of AI" depicts various issues and conflicts that arise in a near-future society where advanced AI has penetrated, in an omnibus format. Along with an introduction to the work, the author explains his ideas and awareness he's put into his work. |
10:40 - 11:00 | Extracting human understanding from mass algorithmic comparison: Case studies in time-series analysis Ben FULCHER, School of Physics, The University of Sydney, Senior Lecturer Abstract: The genetic microarray moved the field of genetics from candidate-gene studies (in which a small selection of genes are tested) to high throughput genome-wide analyses in which thousands of genes and their variations are simultaneously tested, revolutionizing the way we study and understand genetics. Our work has applied a similar philosophy to the selection from amongst thousands of scientific methods for a given analysis task. Instead of a researcher manually selecting an algorithm that may help to, say, detect seizures from EEG data, or uncover the characteristic dynamical signatures of cognition from fMRI data, we advocate for a 'highly comparative' approach in which a comprehensive corpus of possible analysis methods—developed across many decades in diverse scientific disciplines—is searched systematically. This approach overcomes the subjective manual approach to selecting methods for a given problem while also providing human interpretation through the connections to scientific theory underlying each tested algorithm. In this talk I will introduce this approach, the algorithmic libraries that we have developed for univariate and bivariate time-series analysis (our hctsa and pyspi software packages), and some key successes to date. |
11:00 - 11:20 | The Robot Scientist Genesis Ross KING, Chalmers University of Technology, Professor at Data Science and AI, The University of Cambridge, Professor Abstract: A Robot Scientist (AI scientist, self-driving lab) is a physically implemented robotic system that applies techniques from artificial intelligence to execute cycles of automated scientific experimentation. There are now around a hundred Robot Scientist around the world. Most of these systems have two major limitations: 1) They are built to optimise a product, rather than to improve a scientific theory, 2) They do not conclusively demonstrate a cost/time advantage over traditional human science. |
11:20 - 11:40 | Round Table |
11:40 - 12:25 |
Foundation AI models for personalized medicine based on deep human phenotyping Eran SEGAL, Weizmann Institute of Science, Professor Abstract: Recent technological advances allow large cohorts of human individuals to be profiled, presenting many challenges and opportunities. I will present The Human Phenotype Project, a large-scale (>25,000 participants) deep-phenotype prospective longitudinal cohort and biobank that we established, aimed at identifying novel molecular markers with diagnostic, prognostic and therapeutic value, and at developing prediction models for disease onset and progression. Our deep profiling includes medical history, lifestyle and nutritional habits, vital signs, anthropometrics, blood tests, continuous glucose and sleep monitoring, and molecular profiling of the transcriptome, genetics, gut and oral microbiome, metabolome and immune system. Our analyses of this data provide novel insights into potential drivers of obesity, diabetes, and heart disease, and identify hundreds of novel markers at the microbiome, metabolite, and immune system level. Foundation AI models that we developed provide novel representations of the diverse modalities that we measured on the cohort and achieve state-of-the-art performance in predicting future onset of disease and trajectories of disease risk factors. Overall, our predictive models can be translated into personalized disease prevention and treatment plans, and to the development of new therapeutic modalities based on metabolites and the microbiome. |
12:25 - 12:40 | Closing Remark Hiroaki KITANO |
Venue
Facility Name: Nihonbashi Life Science HUB
Location: 1-5-5, Nihonbashi-muromachi, Chuo-ku, Tokyo 103-0022 [Google Map]
Room/Conference Hall: 8F, Conference Room
Door opens at 9:30am
Registration
Registration is required.
Registration will be closed when the number of participants reaches the limit.
Registration Registration Closed
Contact
Workshop Operation Committee
ntci-workshop@oist.jp
The 4th Nobel Turing Challenge Initiative Workshop is organized by
Okinawa Institute of Science and Technology Graduate University (OIST) - Integrated Open Systems Unit, the Systems Biology Institute (SBI), RIKEN, and Nobel Turing Challenge Initiative, co-orgnized by Link-J, with additional support from Corundum Systems Biology Inc. and COI-NEXT
Subscribe to the OIST Calendar: Right-click to download, then open in your calendar application.