FY2019 Annual Report
Neural Coding and Brain Computing Unit
Professor Tomoki Fukai
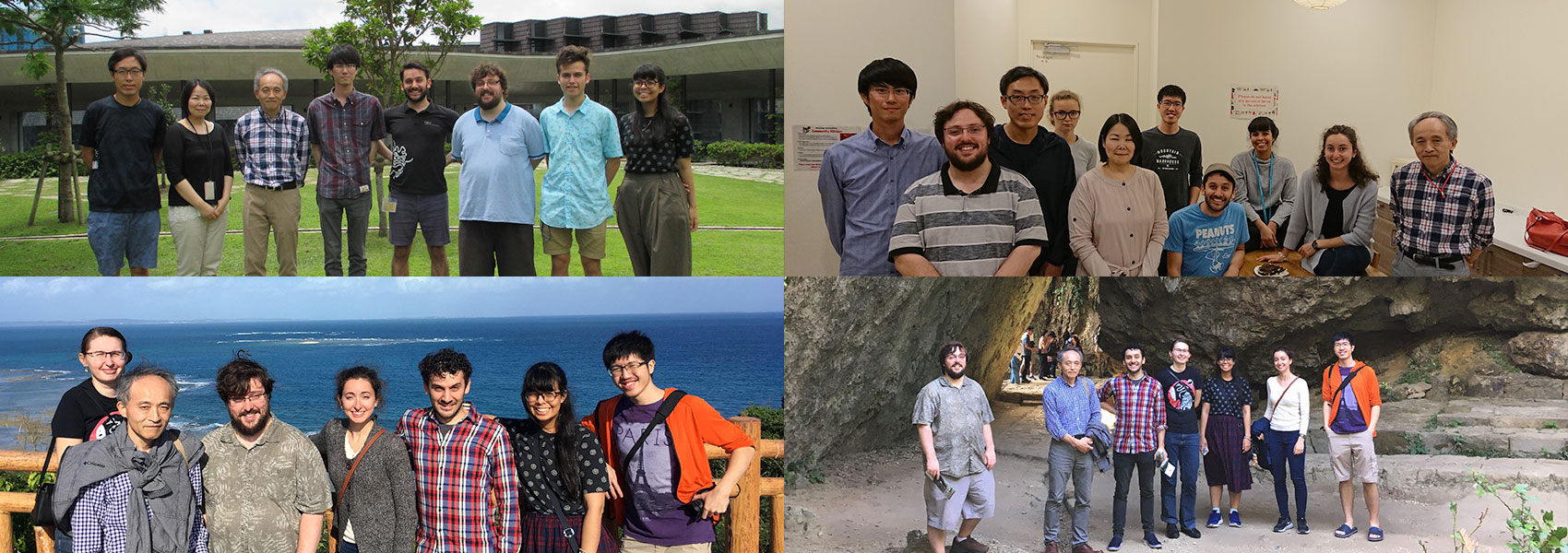
Abstract
Cognitive functions of the brain, such as sensory perception, learning and memory, and decision making emerge from computations by neural networks. We consider that the advantages of biological neural computation in comparison with machine computation reside in the way that the brain’s neural circuits implement computation. To uncover neural code and circuit mechanisms of brain computing, we take computational and theoretical approaches. Our goal is to construct a minimal but yet effective description of powerful and flexible computation implemented by the brain’s neural circuits.
1. Staff
- Staff Scientist
- Chi Chung Fung, PhD
- Postdoctoral Scholar
- Ibrahim Alsolami, PhD
- Hongjie Bi, PhD
- Research Unit Technician
- Ruxandra Cojocaru, PhD
- PhD Student
- Thomas Burns
- Gaston Sivori
- Maria Astrakhan
- Special Research Student
- Toshitake Asabuki
- Milena Menezes Carvalho
- Rotation Student
- Gaston Sivori (Term 3)
- Maria Astrakhan (Term 1)
- Loh Chen Lam (Term 1)
- Roman Koshkin (Term 2)
- Florian Lalande (Term 2)
- Research Intern
- Joshua Stern (May-Aug 2019)
- Giorgia Dellaferrera (Oct-Dec 2019)
- Hugo Musset (Feb-Jul 2020)
- Research Unit Administrator
- Kiyoko Yamada
2. Collaborations
2.1 "Idling Brain" project
kaken.nii.ac.jp/en/grant/KAKENHI-PROJECT-18H05213
- Supported by Grant-in-Aid for Specially Promoted Research (KAKENHI)
- Type of collaboration: Joint research
- Researchers:
- Professor Kaoru Inokuchi, University of Toyama
- Dr. Khaled Ghandour, University of Toyama
- Dr. Noriaki Ohkawa, University of Toyama
- Dr. Masanori Nomoto, University of Toyama
2.2 Chunking sensorimotor sequences in human EEG
- Type of collaboration: Joint research
- Researchers:
- Professor Keiichi Kitajo, National Institute for Physiological Sciences
- Dr. Yoshiyuki Kashiwase, OMRON Co. Ltd.
- Dr. Shunsuke Takagi, OMRON Co. Ltd.
- Dr. Masanori Hashikaze, OMRON Co. Ltd.
2.3 Cell assembly analysis in the rodent motor cortex
- Type of collaboration: Joint research
- Researchers:
- Professor Masami Tatsuno, the University of Lethbridge
2.4 Cell assembly analysis in the songbird brain
- Type of collaboration: Internal joint research
- Researchers:
- Professor Yoko Yazaki-Sugiyama, OIST
- Dr. Jelena Katic, OIST
3. Activities and Findings
3.1 Temporal feature segmentation and its implementation with dendritic computing
https://www.nature.com/articles/s41467-020-15367-w
How does the brain identify potentially salient features within continuous information streams? In the novel solution we proposed to this fundamental problem of brain computing, we implemented a self-supervising learning process in a two-compartment neuron model with dendritic structure. This model learns repeated clustered temporal events in an unsupervised manner by minimizing information loss between dendritic synaptic input and a neuron’s own output spike trains. The resultant learning rule conceptually resembles Hebbian learning with backpropagating action potentials, which experimental results have demonstrated to be crucial for synaptic plasticity in cortical neurons. A family of networks composed of the two-compartment neurons performs a surprisingly wide variety of complex unsupervised learning tasks, including chunking of temporal sequences and the source separation of mixed correlated signals. Our results suggest the powerful ability of neural networks with dendrites to analyze temporal features. In addition, the proposed neuron model is potentially useful in neural engineering applications such as neuromorphic computing.
Figure 1: A family of competitive networks of two-compartment neurons (top left). The network performs multiple temporal features analyses such as sequence chunking (top right) and blind source decomposition (bottom).
3.2 Mean-field theory for strongly balanced recurrent networks
https://journals.aps.org/prresearch/abstract/10.1103/PhysRevResearch.2.013253
Neural dynamics with balanced excitation-inhibition play a pivotal role in information processing by the brain. Previous theories of brain dynamics were restricted to an inhibition dominant regime in which fluctuations in the macroscopic population activity are strongly suppressed. In this study, we investigated the dynamics of neural networks at a critical point between excitation-dominant and inhibition-dominant states. We found that such networks exhibit nontrivial interscale interactions in which the microscopic fluctuations in neuronal activity amplified by strong excitation and inhibition drive the macroscopic network dynamics, while the macroscopic dynamics determine the statistics of the microscopic fluctuations. As a consequence of the amplification mechanism, the network models generated spontaneous, irregular macroscopic rhythms similar to those observed in the brain. To analytically study the interscale interactions, we developed a novel type of mean-field theory. Our results suggest a novel form of neuronal information processing that connects neuronal dynamics on different scales.
3.3 Inhibitory circuit regularizable temporal association memory
https://journals.aps.org/prl/abstract/10.1103/PhysRevLett.123.078101
Hebb postulated that synchronous activation strengthens connections between neurons in the brain, and these strongly connected neuron ensembles are the basis of associative memory. Today, Hebb’s postulate is a widely accepted paradigm for memory processing in the brain. Hebbian learning suggests an attractor network model that can recall activity patterns from incomplete external cues. While Hebbian learning is sufficient for supporting the attractors, the role of inhibitory modulation for associative memory remains unclear. In a class of attractor network models that stores sequentially presented items in spatially correlated attractors, we showed that Hebbian learning combined with anti-Hebbian learning of inhibitory synapses can significantly increase the span of the temporal association between correlated attractors. Interestingly, the ratio of local and global recurrent inhibition can regulate this effect. Our results suggest a previously unknown role of inhibitory circuits in the temporal clustering of episodic events. This work was introduced in the following news articles:
OIST Research News
RIKEN Research News
Figure 2: A temporal association network with global and local inhibition (top left) has correlated attractors with a modifiable temporal span (top right). Oscillatory modulations of global vs local inhibition (middle) can shift the central pattern of correlated attractor states (bottom).
Figure 3: Non-negative matrix factorization of neural data (top). Two example sets of cell-assembly patterns and the corresponding occurrence matrices are shown (middle). Reactivation of cell assembly patterns was investigated for different task phases and days (bottom).
3.4 Orchestration of hippocampal memory engram cells
https://www.nature.com/articles/s41467-019-10683-2
The brain stores and recalls memories through a set of neurons termed engram cells. However, it is unclear how these cells are organized to constitute a corresponding memory trace. In collaboration with experimentalists at Toyama University Medical School, we analyzed the Ca2+ imaging data recorded with a novel technique to identify hippocampal engram activity. We used a mathematical tool called “non-negative matrix factorization” combined with Akaike information criteria. Our analysis revealed the collective activation of several sub-ensembles of neurons during learning. Furthermore, some sub-ensembles preferentially reappeared during post-learning sleep, and these replayed sub-ensembles were more likely to be reactivated during retrieval. These results indicate that sub-ensembles represent distinct pieces of information, which are then orchestrated to constitute an entire memory.
3.5 Edit similarity for cell-assembly detection
https://www.frontiersin.org/articles/10.3389/fninf.2019.00039/full
Neurons that fire in a fixed temporal pattern, i.e., cell assemblies, are hypothesized to be a fundamental unit of neural information processing. However, the systematic detection of cell assemblies with varying time structure has been challenging. Here, we developed a novel method to detect a variety of cell-assembly activity patterns, recurring in a noisy neural population. The key innovation is the use of a computer science method to comparing strings (“edit similarity”), to group spikes into assemblies. We applied this method to multi-neuron activity data recorded from the hippocampus and prefrontal cortex of behaving animals. We showed that the method offers a powerful analytical tool for studying the neural code represented by cell-assembly activation patterns.
4. Publications
4.1 Journals
- Toshitake Asabuki and Tomoki Fukai (2020) Somatodendritic consistency check for temporal
feature segmentation. Nat Commun, 11, 1554, doi:10.1038/s41467-020-15367-w - Takashi Hayakawa and Tomoki Fukai (2020) Spontaneous and stimulus-induced coherent states of critically balanced neuronal networks. Phys. Rev. Research, 2, 013253, doi:10.1103/PhysRevResearch.2.013253
- Khaled Ghandour, Noriaki Ohkawa, Chi Chung Alan Fung, Hirotaka Asai, Yoshito Saitoh, Takashi Takekawa, Reiko Okubo-Suzuki, Shingo Soya, Hirofumi Nishizono, Mina Matsuo, Makoto Osanai, Masaaki Sato, Masamichi Ohkura, Junichi Nakai,Yasunori Hayashi, Takeshi Sakurai, Takashi Kitamura, Tomoki Fukai and Kaoru Inokuchi (2019) Orchestrated ensemble activities constitute a hippocampal memory engram. Nat Commun, 10, 2637, doi:10.1038/s41467-019-10683-2
- Tatsuya Haga and Tomoki Fukai (2019) Extended temporal association memory by modulations of inhibitory circuits. Phys Rev Lett, 123, 078101, doi:10.1103/PhysRevLett.123.078101
- Keita Watanabe, Tatsuya Haga, Masami Tatsuno, David R Euston and Tomoki Fukai (2019) Unsupervised detection of cell-assembly sequences by similarity-based clustering. Front Neuroinform, 13(39): 1-19, doi: 10.3389/fninf.2019.00039
4.2 Books and other one-time publications
- Tomoki Fukai (2019) Susceptibility of neural trajectory evolution explains behavioral variances in decision making (Japanese). KAKENHI Project (AI & Brain Science) News Letter, vol. 6: 20-21.
http://www.brain-ai.jp/wp-content/uploads/2019/11/newsletter06.pdf - Tomoki Fukai (2019) Brain's computing versus AI's (Japanese). Journal of Human Life Engineering, vol. 20, no. 2: 1-4.
4.3 Oral and Poster Presentations
Oral Presentations
- Tomoki Fukai, Dendrites as an essential component of brain computing, 新学術領域研究 オシロロジー・ 人工知能と脳科学・脳情報動態 合同シンポジウム「脳型計算アーキテクチャ」, Tokyo, Japan (December 2019)
- Tomoki Fukai, Cognition through neural circuit dynamics, Toyama Forum for Academic Summit on "Dynamic Brain", Toyama, Japan (December 2019)
- Tomoki Fukai, Temporal feature analysis in brain-inspired neural systems, Silicon Nanoelectronics Workshop 2019, Kyoto, Japan (June 2019)
- Tomoki Fukai, Extended temporal association memory by modulations of inhibitory circuits, The 6th Research Area Meeting "Artificial Intelligence and Brain Science", Tokyo, Japan (May 2019)
- Tomoki Fukai, Temporal feature analysis by self-supervising dendritic neurons, 2019 Gordon Research Conference on Dendrites: Molecules, Structure and Function, Ventura, USA (April 2019)
Poster Presentations
- Tomoki Fukai, Learning complex temporal features by neurons with dendrites, OIST-Hitachi Joint Symposium, Tokyo, Japan (February 2020)
- Thomas Burns, Tomoki Fukai, Stability of spontaneous activity depends on synaptic delays in spiking network models, Systems & Computational Neuroscience Down Under (SCiNDU) 2020, Brisbane, Australia (January 2020)
- Tatsuya Haga, Tomoki Fukai, Multiscale associative memory recall by modulation of inhibitory circuits, 新学術領域研究 オシロロジー・ 人工知能と脳科学・脳情報動態 合同シンポジウム「脳型計算アーキテクチャ」, Tokyo, Japan (December 2019)
Other
- Tomoki Fukai, guest panel speaker, Bias-Free Life Science- How can newly emerged technologies illuminate the reality of life? -, The 42nd Annual Meeting of the Molecular Biology Society of Japan, Fukuoka, Japan (December 2019)
5. Intellectual Property Rights and Other Specific Achievements
5.1 Grants
Kakenhi Grant-in-Aid for Scientific Research on Innovative Areas (Research in a proposed research area)
- Titile: Creation of novel paradigms to integrate neural network learning with dendrites
- Period: FY2019-2020
- Principal Investigator: Prof. Tomoki Fukai
Kakenhi Grant-in-Aid for Specially Promoted Research
- Titile: Mechanisms underlying information processing in idling brain
- Period: FY2018-2022
- Principal Investigator: Prof. Kaoru Inokuchi (University of Toyama)
- Co-Investigators: Prof. Tomoki Fukai, Prof. Keizo Takao (University of Toyama)
Kakenhi Graint-in-Aid for Early-Career Scientists
- Titile: A Comprehensive Theoretical Survey on Functionalities and Properties of Adult Neurogenesis under the Influence of Slow Oscillations
- Period: FY2019-2022
- Principal Investigator: Dr. Chi Chung Fung
6. Meetings and Events
6.1 Seminars
Deep Turning Point
- Date: September 3, 2019
- Venue: OIST Campus Lab1, C016
- Speaker: Dr. Danilo Vasconcellos Vargas (Kyushu University)
Multiscale associative memory recall by modulation of inhibitory circuits
- Date: February 5, 2020
- Venue: OIST Campus Lab1, D014
- Speaker: Dr. Tatsuya Haga (RIKEN Center for Brain Science)
6.2 Events
2019 Team Meeting of Grant-in-Aid for Specially Promoted Research - Mechanisms underlying information processing in idling brain -
- Date: April 22-23, 2019
- Venue: OIST Conference Center, Meeting Room 3
- Co-organizer: Prof. Kaoru Inokuchi (University of Toyama)
OIST Computational Neuroscience Course 2019
- Date: June 24- July 11, 2019
- Venue: OIST Seaside House
- Organizers: Erik De Schutter (OIST), Kenji Doya (OIST), Tomoki Fukai (OIST), Bernd Kuhn (OIST), and Jeff Wickens (OIST)
Skill Pills Plus Workshop: Neural Coding and Brain Computing
- Date: November 8-10, 2019
- Venue: OIST campus
- Organizer: OIST Graduate School
7. Other
Program Committee, Annual Computational Neuroscience Meeting (CNS2019)