"Machine Learning: Making Complex Data Analysis Possible" Dr. Michael Gutmann
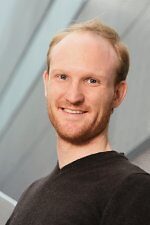
Date
Location
Description
Dr. Michael Gutmann
Postdoctoral fellow
Department of Mathematics and Statistics
University of Helsinki, Finland
Abstract
As scientists, we are often confronted with the analysis of complex and noisy data. Statistical modeling and inference provide the fundamental principles behind data analysis. But for complex models and data sources, the computational cost of implementing the principles and performing inference exactly is prohibitively large.
In the talk, I first give an overview of my research on approximate yet computationally feasible inference. This includes machine learning methods which we developed to answer questions arising in visual object recognition. Then I present recent work on individual-based models simulating the spread of infectious diseases. I explain how machine learning has enabled more complex data analysis than ever before by reducing the computational cost of inference by factors of 1000 or more.
Biography
Michael Gutmann is a postdoctoral fellow at the Department of Mathematics and Statistics at the University of Helsinki, Finland. He studied engineering and applied mathematics at the Swiss Federal Institute of Technology (ETH) Zurich and the French engineering school Ecole Centrale Paris. He obtained his PhD in computational neuroscience from the University of Tokyo in 2008. His current research interests include statistical inference for complex models, unsupervised deep learning, natural image statistics, computational neuroscience, and infectious disease epidemiology.
Link to publication: https://sites.google.com/site/michaelgutmann/publications
Subscribe to the OIST Calendar: Right-click to download, then open in your calendar application.