[Seminar] MLDS Unit Seminar 2024-6 by Mr. Kazusato Oko (The University of Tokyo), Mr. Yuki Takezawa (Kyoto University) at C210
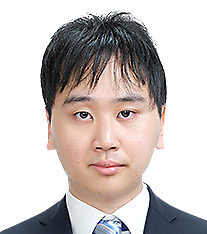
Date
Location
Description
Speaker 1: Mr. Kazusato Oko (The University of Tokyo)
Title: How to learn a sum of diverse features provably: A case study of ridge combinations
Abstract: In the paradigm of foundation models, neural networks simultaneously acquire diverse features for different tasks during pretraining. The learned features are often localized in distinct parts of the trained network, allowing fine-tuning to easily select a few of them for each downstream task. Motivated by this, we aim to rigorously analyze the learning dynamics of ridge combinations with a two-layer neural network, that cannot be efficiently learned without localization of features in different neurons. Specifically, the target function $f_*:\mathbb{R}^d\to\mathbb{R}$ has an \textit{additive structure}, that is, $f_*(x) = \frac{1}{\sqrt{M}}\sum_{m=1}^M f_m(\langle x, v_m\rangle)$, where $f_1,f_2,...,f_M:\mathbb{R}\to\mathbb{R}$ are nonlinear link functions of single-index models (ridge functions) with diverse and near-orthogonal index features $\{v_m\}_{m=1}^M$ and the number of additive tasks $M$ grows with the dimensionality $M\asymp d^\gamma$ for $\gamma\ge 0$. We show that a large subset of polynomial $f_*$ can be efficiently learned by gradient descent training of a two-layer neural network, with a polynomial statistical and computational complexities that depend on the number of tasks $M$ and the \textit{information exponent} of $f_m$, despite the unknown link function and $M$ growing with the dimensionality.
This talk is based on joint work with Yujin Song, Taiji Suzuki, and Denny Wu.
Speaker 2: Mr. Yuki Takezawa (Kyoto University)
Title: Polayk Meets Parameter-free Clipped Gradient Descent
Abstract: Gradient descent and its variants are de facto standard algorithms for training machine learning models. As gradient descent is sensitive to its hyperparameters, we need to tune the hyperparameters carefully using a grid search, but it is time-consuming, especially when multiple hyperparameters exist. Recently, parameter-free methods that adjust the hyperparameters on the fly have been studied. However, the existing work only studied parameter-free methods for the stepsize, and parameter-free methods for other hyperparameters have not been explored. For instance, the gradient clipping threshold is also a crucial hyperparameter in addition to the stepsize to prevent gradient explosion issues, but none of the existing studies investigated the parameter-free methods for clipped gradient descent. In this work, we study the parameter-free methods for clipped gradient descent. Specifically, we propose Inexact Polyak Stepsize, which converges to the optimal solution without any hyperparameters tuning, and its convergence rate is asymptotically independent of $L$ under $L$-smooth and $(L_0, L_1)$-smooth assumptions of the loss function as that of clipped gradient descent with well-tuned hyperparameters. We numerically validated our convergence results using a synthetic function and demonstrated the effectiveness of our proposed methods using LSTM, Nano-GPT, and T5.
Subscribe to the OIST Calendar: Right-click to download, then open in your calendar application.