[ONOS Seminar Series] Professor. Alexander Mathis: Modeling sensorimotor circuits with task-driven & reinforcement learning
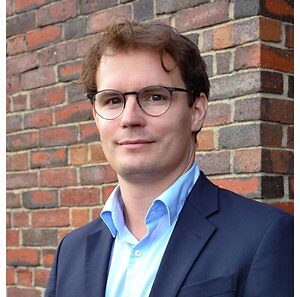
Date
Location
Description
Adaptive motor control crucially depends on proprioception and hierarchical control. I will present two recent lines of work that seek to gain insights into these topics. Firstly, I will present a task-driven neural network modeling approach to quantitatively test hypotheses about the functional role of proprioceptive neurons (Sandbrink*, Mamidanna* et al., eLife 2023). Based on this framework, we tested different tasks as hypotheses about the function of the ascending proprioceptive pathway by predicting neuronal spiking activity from macaques performing a center-out reaching task (Marin Vargas* & Bisi* et al. Biorxiv 2023). To do so, we tracked limb movements using DeepLabCut and inferred the proprioceptive signals via musculoskeletal modeling. We used these as inputs to the task-trained DNNs to linearly regress single-neuron activity. We found that models that perform better on synthetic proprioceptive task, generalize better to explain neural data. These results suggest that these tasks are sufficient to develop brain-like representations along the proprioceptive pathway. Secondly, I will discuss DMAP, a biologically-inspired, attention-based policy network architecture (Chiappa et al., NeurIPS 2022). It combines a distributed policy, with individual controllers for each joint, and an attention mechanism, to dynamically gate sensory information from different body parts to different controllers. We study DMAP in four classical continuous control environments, augmented with morphological perturbations. Learning to locomote when the length and the thickness of different body parts vary is challenging, as the policy is required to adapt to the morphology to successfully balance and advance the agent. We show that a control policy based on the proprioceptive state performs poorly with highly variable body configurations, while an (oracle) agent with access to a learned encoding of the perturbation performs significantly better. We find that DMAP can be trained end-to-end in all the considered environments, overall matching or surpassing the performance of an oracle agent with access to the (hidden) morphology information. Thus DMAP, implementing principles from biological motor control, provides a strong inductive bias for learning challenging sensorimotor tasks. Lastly, I will discuss how we have started to combine representation and reinforcement learning for musculoskeletal control.
Subscribe to the OIST Calendar: Right-click to download, then open in your calendar application.