FY2023 Annual Report
Biological Nonlinear Dynamics Data Science Unit
Assistant Professor Gerald Pao
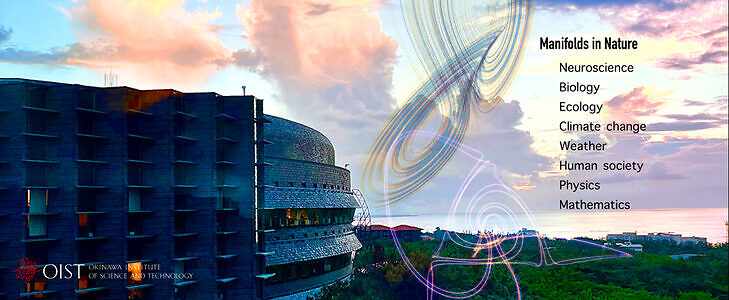
Abstract
In the past year we were in the process of establishing the lab. Reagents from La Jolla California arrived in August 2023 and equipment arrived in November 2023.
Staff Scientist Junko Ogawa established the cell culture and microscopy capabilities for time series collection from live microcopy.
Group leader Joseph Park arrived from the United nations in Vienna in February 2024 but due to a lack of on campus housing and an age cap of 65 he moved back to Florida to work remotely. He is currently working on causal compression, dimensionality reduction technique that allows experimental verification that we consider a cornerstone of a new paradigm to do data driven science as opposed to the traditional hypothesis driven science. This is a paradigm shift from traditional science that takes advantage of big data and computational capability that was hitherto not available.
Yaroslav Korobov is a new student and joined the lab. He will be working methods for the discovery of data geometry for edge cases where current techniques fail within the empirical dynamic modeling framework.
1. Staff
- Dr. Gerald Pao, Assistant Professor
- Dr. Junko Ogawa, Staff Scientist
- Joseph Park, Group Leader
- Yaroslav Koborov, PhD student
- Ms. Kayoko Ikeda, Research Unit Administrator
<Rotation Students>
- Dmitriy Sakharuk (Sept-Dec 2023)
- Kota Shirahata (Sept-Dec 2023)
- Tatsunosuke Hanano (Sept-Dec 2023)
- Clea Mehnia Laouar (Jan-Mar 2024)
- Diana Nechepurenko (Jan-Mar 2024)
- Tamara Iakimova (Jan-Mar 2024)
2. Collaborations
2.1 Decision making in isolated brains.
- Description: Using ex vivo isolated brains from Tritonia spp. and Aplysia californica sea snails, The Frost lab at Rosalind Franklin University has recorded from the entire suface of the brain using fluorescent probes. We are currently collaborating with the Frost lab to elucidate the neural mechanisms of decision making in solated ex vivo brains that can undergo fictive motor output decisions in response to electrophysiological stimulation designed to simulate sensory input.
- Type of collaboration: Joint research
- Researchers:
- Professor William Frost, Rosalind Franklin University
- Professor Jeffrey Brown, Penn State University
2.2 Whole brain sensorimotor integration at single neuron resolution
- Description: Establishing the information sharing pathways of a whole vertebrate at single neuron resolution and how sensorimotor integratoin is generated in a vertebrate brain by neurons and glia.
- Type of collaboration: Joint research
- Researchers:
- Dr. Misha Ahrens, Senior group leader, Janelia Research Campus HHMI
- Dr. First Last, Another University
2.3 Whole brain body communication
- Description: Using causal inference to establish the brain body interactions of a whole organism.
- Type of collaboration: Joint research
- Researchers:
- Dr. Misha Ahrens, Senior group leader, Janelia Research Campus HHMI
2.4 Noise and turbulence analysis in microfluidic devices
- Description: Using EDM based data sceince to analyze whether fluctuations observed in microfluiidc devices are from noise or turbulence
- Type of collaboration: Joint research
- Researchers:
- Professor Amy Shen OIST
- Dr. Ricardo Lopez OIST
2.5 Mapping low density neural recordings to physiology in Zebrafinches
- Description: Attempting to generate a mathematical model to partially infer neural activity based on physiolocal observable parameters.
- Type of collaboration: Joint research
- Researchers:
- Professor Yoko Yazaki Sugiyama OIST
- Dr. Kauthar Samarat OIST
- Yaroslav Korobov OIST
- Dr. Junko Ogawa OIST
2.6 Reflectin gene diversity and Evolution across cephalopods
- Description: Cloning and characterization of reflectin genes across cephalopods to understand the evolutionary history of reflectins and their physico-chemical properties.
- Type of collaboration: Joint research
- Researchers:
- Professor Jonathan Miller OIST
- Dr. Zdenek Ljabner OIST
- Dr Lucia Zivkakova OIST
- Dr. Junko Ogawa OIST
- Dr. Atsushi Miyawaki, RIKEN CBS
- Dr. Yoko Iwata, U of Tokyo
2.7 Mathematical principles underlying the relationship between high dimensional spaces and low dimensional solutions in learning representations
- Description: Using EDM and new mathematical objects to describe learning at every scale both in artificial neural networks and brains.
- Type of collaboration: Joint research
- Researchers:
- Professor Terrence Sejowski Salk/UCSD
- Profesosr Stanislav Smirnov, University of Geneva
- Dr. Alessandra Camassa
- Dr. Joseph Park
2.8 Detecting early warning signs of critical transitions and interventions through normal physoiological aging.
- Description: Using EDM based data science approaches to analyze physiological aging treatign aging as an anomaly for which we can see the early signs where we can ameiorate the imapct of aging in humans.
- Type of collaboration: Joint research
- Researchers:
- Professor Caroline Wee, Astar/NUS
- Professor Weiping Han, Astar
- Professor Sarah Xinwei Luo, Astar
- Professor Rosa Qiyue So, Astar
- Dr. Mengjiao Hu, Astar
- Dr. Junko Ogawa, OIST
2.9 Whole brain sesorimotor deciion making itegration in the rodent brain
- Description: Using EDM based data science to integrate recordings from multiple brain areas to may brain activity to behavior on the surfaces of low dimensional nonlinear manifolds.
- Type of collaboration: Joint research
- Researchers:
- Professor Loren Frank UCSF/HHMI
- Dr. Shih-Yi Tseng UCSF
2.10 Brain machine interfaces for human driving
- Description: Using EDM based data science to analyze whether fluctuations observed in microfluiidc devices are from noise or turbulence
- Type of collaboration: Joint research
- Researchers:
- Professor Jack Gallant, UC Berkeley
- Dr. Tianjiao Zhang, UC Berkeley
2.11 Scalable high perfomance computing (HPC) for EDM and other manifold learning algorithms
- Description: Many algorithms for dimensionality reduction including EDM, MIND and others are computationally expensive and becasue of that in many cases not scalable. Wtih profesor Takahashi we have had an ongoing project to continually update EDM algorithms to make them adaptable to the newest hardware that the HPC community uses so that our software performs optimally in hardware supported by theb Kokkos ecosystem.
- Type of collaboration: Joint research
- Researchers:
- Professor Keichi Takahashi, Tohoku University
- Dr. Joseph Park, OIST
2.12 Visualization tools for EDM
- Description: EDM based data science frequently works in dimensions greater than 3 and can be up to 10 dimensions in many cases. As such humans cannot visualize such objects directly. In order to deal with data exploration of data with dimensions greater than 3 in collaboration with professor Hiroaki Natsukawa we have a program to generate a visualization envirnment that helps researchers understand their data in a visual way in 3 dimensions or less but cand evelop intuition of 3 or more dimensions.
- Type of collaboration: Joint research
- Researchers:
- Professor Hiroaki Natsukawa, Osaka Seikei University
- Dr. Joseph Park, OIST
3. Activities and Findings
In the last year we have made two major findings.
The first one is technical in nature and the second is both technical and scientific.
The first significant advance is the creation of a new dimensionality reduction method called Causal Compression, which uses Takens theorem based causal inference of dynamical systems to generate reduced dimensional representations that have no mathematical abstraction that don’t have 1:1 correspondence to real world. A prime and most popular example of this is Principal component analysis where the 1. Principal component (PC1) is a vector in the direction of greatest variance of the cloud of points that are the totality of the data with the magnitude of the vector being the projection of every data point in the direction of PC1. If we use brain activity as an example, this could the 20% on neuron 1, 50% of neuron 2, 5% of neuron 3 …. Etc. But if we were to try do an experiment what does it mean to manipulate 20% on neuron 1, 50% of neuron 2, 5% of neuron 3 …. Etc? Assuming that we want to an experiment to confirm the relevance of PC1 we would need to manipulate 20% on neuron 1, 50% of neuron 2, 5% of neuron 3 …. etc. We can either manipulate a neuron or not manipulate it but we have not capacity to manipulate fractional amounts of neuron. This is this an impossibility to manipulate any PC in principle. As far as we know every dimensionality reduction commonly used suffers from this problem.
To solve this problem we have created a new algorithm that generates experimentally testable hypotheses where every component or axis corresponds to a real measured feature, Thus in this manner the relationships derived from this method not only correspond to real observables, which makes it more intuitive but can also be experimentally verified which makes the method particularly useful for experimentalists.
The method entails finding the cause and effect relationships within a dataset using convergent cross mapping, a causal inference algorithm and then within the causal factors identified we combine multiple candidate causal factors so as that they are complementary to each other in their ability to predict a particular variable that we are interested in. We stop when we do not find anymore variables that can help us predicting better as we add additional variables from the ones we have previously identified. The result is a geometric shape called manifold that allows us to establish the relationship between all the relevant variables and at the same time it allows us to do prediction of future behaviors of the system that we are interested in. We named this method causal compression and we are in the process of developing a software package to formalize the procedure and make it generally available to the scientific community.
Our second major finding is on climate change and it also came as a consequence of the development of another new algorithm. The algorithm we developed was in response to a technical limitation of a mathematical technique named time delay embedding, or more generally lagged coordinate embedding or Takens embeddings, a mathematical method invented by 4 UC Santa Cruz physics students and proven subsequently by Floris Takens. Takens embeddings are equation free geometrical models that allow predictions of future behaviors of a system as well as extracting various properties such as complexity of the system etc. However as traditionally formulated by the original proof of Takens in 1981, Takens embedding have a characteristic time scale to produce analyses or predictions and fundamentally cannot deal with processes that occur at multiple time scales. An example of this is that if we want to predict behaviors that occur in daily cycles, we will not be able to study seasonally or yearly cycles. Thus this becomes a limitation as we have to commit to a particular time scale of study. Furthermore we will not be able to know how seasonal processes will affect daily or yearly cycles, i.e. we cannot have multiscale descriptions. To solve this problem we took advantage of an extension of the Takens theorem, the generalized Takens theorem proven in 2011. The generalized Takens theorem extends the Takens theorem in some key ways in that it allows for the mix of multiple real variables and delays, uneven sampling and most importantly for our case irregular time delays. The use of irregular time delays makes it possible to incorporate processes that occur at different time scales into a single embedding. In this manner occurrences at additional time scales of interest are incorporated as additional dimensions within the same embedding. This is particularly useful if the system for which we do not have all the variables but where dynamics occur at multiple timescales has to be modeled. An additional feature is that a single system can also be modeled not with a single manifold but with a whole population of manifolds with differing time delays. This use of every possible time scale is useful for the detection of anomalies in data that have no historical precedent. Usually a modeled has to be tuned for a particular state but if a new state appears, it will be by definition unprecedented and unlike anything observed before. Therefore embeddings that a rely on particular delays will likely not be optimal for the detection of the appearance of unprecedented variables. Thus a comprehensive collection of embeddings at every time scale within a reasonable range for the system in question can resolve this. Having every possible projection is bound to include one or more embeddings that are suitable for anomaly detection of a new variable even if we did not have prior knowledge of any type about the novel factor or occurrence. In the context of climate Change we have discovered that the Antarctic Sea Ice cycle and the global sea surface temperature have undergone state changes since May of 2023. We are in the process of trying to determine if this change is a change from a buffered system that has negative feedback to one of positive feedback.
4. Publications
4.1 Journals
-
K. Takahashi, K. Ichikawa, J. Park and G.Pao, "Scalable Empirical Dynamic Modeling With Parallel Computing and Approximate k-NN Search," in IEEE Access, vol. 11, pp. 68171-68183, 2023, doi: 10.1109/ACCESS.2023.3289836.
-
J Park, G Sugihara, G Pao.(2023) Control of complex systems with generalized embedding and empirical dynamic modeling. arXiv preprint arXiv:2311.17324, 2023
4.2 Books and other one-time publications
Toward Scalable Empirical Dynamic Modeling
K Takahashi, K Ichikawa, GM Pao
Sustained Simulation Performance 2022
Joint Workshop on Sustained Simulation Performance, 61-69
4.3 Oral and Poster Presentations
([NOTE] *Seminars and workshops by OIST faculty/unit members (either with or without other speakers), either at OIST or at other institutions than OIST, should be included in the 4.3 Oral and Poster Presentations.
-
Gerald Pao, Selected Tutorial: Introduction to empirical dynamic modeling: A suite of dynamical causal inference methods
Conference "Computational & Theoretical Zebrafish Neuroscience" at HHMI Janelia Research Campus, Virginia USA, April 23-26, 2023 - Gerald Pao, Talk: Experimentally testable whole brain manifold networks that recapitulate behavior and neural dynamics
Conference "Computational & Theoretical Zebrafish Neuroscience" at HHMI Janelia Research Campus, Virginia USA, April 23-26, 2023
- Gerald Pao, The intrinsic geometry of brain activity: From single neurons to whole brains
Charles F. Stevens Conference at Salk Institute, California USA, May 3-5 2023
-
Gerald Pao, Talk: "An algorithm to generically map neural activity to behavior” (Where we described a new dimensionality reduction framework based on causal inference that has no latent variables and in this way allows for the experimental verification of hypotheses which is not possible with the prevalent methods in existence.)
2023 Woods Hole Workshop on Computational Neuroscience (from July 2-9) which is the middle week of the 2023 Telluride Neuromorphic Cognition Engineering Workshop from June 25 - July 14, 2023, in Telluride, Colorado, USA, an NSF sponsored workshop since 1994 established by Carver Meade to investigate applications and designs of neuromorphic chips. These are computer chips that are inspired by animal brains where computers chips spike instead of holding constant current and are therefore more similar to real animal brains and use vastly less energy to run.
For a complete program see below link
https://sites.google.com/view/telluride-2023/topic-areas/cns23-woods-hole-workshop-on-computational-neuroscience?authuser=0 -
Gerald Pao Talk at Okinawa Comp Neuro course
Empirical dynamic modeling: manifold methods lectures. July 29 2023. -
Gerald M Pao, Talk “Mapping brain activity to behavior on the surface of low dimensional manifolds”. OIST-RIKEN Brain Symposium, August 21, 2023. OIST, Japan.
-
Poster presentation “Widespread presence of low dimensional manifolds across the Zebrafish brain at single neuron resolution“. Alessandra Camassa, Hiroaki Natsukawa, Ziqiang Wei, Joseph Park, Chen Min Yeh, Sreekanth Chalasani, Misha B Ahrens, Terrence J Sejnowski, Gerald M Pao.
Lake Conference – Neural Coding and Dynamics. September 17 - 21, 2023. -
Gerald Pao, OIST Board of Governors talk, September 28th 2023.
-
Gerald Pao and Alessandra Camassa: EDM Tutorial Session, October 18th, 2023. RIKEN, Saitama, Japan.
-
Gerald Pao“Causation without correlation in Biology “, The 164th Science-ome (Online).October 25, 2023.
-
Gerald Pao, Scripps Institution of Oceanography, UCSD
SIO 278 class "Empirical dynamic modeling case studies: science beyond natural human intuition" November 9, 2023. -
Poster presentation “Causal relationships between brain areas across primate evolution”
Junko Ogawa, Hao Ye, Shyam Srinivasan, Charles F. Stevens, George Sugihara and Gerald M. Pao. Society for Neuroscience, 2023. November 14, 2023. Board B50 at the Walter E. Washington Convention Center, Washington, DC. -
Poster presentation “Low dimensional manifolds of whole brain activity at single neuron resolution that map activity to behavior”
Joseph Park, Alessandra Camassa, Cameron Smith, Hiroaki Natsukawa, Ziqiang Wei, Chen Min Yeh, Sreekanth Chalasani, Sophie Aimon, Misha B Ahrens, Terrence J Sejnowski, Gerald M Pao. Society for Neuroscience, 2023. November 14, 2023. Board XX44 at the Walter E. Washington Convention Center, Washington, DC. -
Gerald M.Pao, Invited talk “The intrinsic Geometry of Data and what it can tell us”
at Astar, Singapore. November 29, 2023. -
Gerald Pao, Presentation "An algorithm to generically map neural activity to behavior", December 4, 2023. University of California, Santa Cruz, CA, USA.
-
Gerald Pao, Presentation "An algorithm to generically map neural activity to behavior", December 5, 2023. University of California, San Francisco, CA, USA.
-
Gerald Pao, Presentation "Takens embeddings for multiple time scales", December 11, 2023. AGU (American Geophysical Union) 23, in San Francisco, CA, USA.
-
Gerald Pao, Talk “Extreme Nonlinearity and Causation without correlation”
RIKEN-OIST Prediction workshop , December 21 2023. OIST, Japan. -
Gerald Pao, Talk at Cabinet office meeting OIST January 19, 2024.
“Early warning signs of catastrophic transitions and causal inference from satellite data” -
Gerald Pao, “Manipulation of the optical refractive index of mammalian cells in vivo with genetically encoded cephalopod Reflectin proteins”
January 19, 2024 JST-OIST Macromolecular Assembly workshop at OIST. -
Gerald Pao, Lecture at UCSD Winter 2024 AI and Brains, "Downloading a Brain With Convergent Cross Mapping", February 2, 2024. University of California, San Diego, CA, USA.
-
5. Intellectual Property Rights and Other Specific Achievements
Generative manifold networks for prediction and simulation of complex systems
G Pao, C Smith US Patent App. 18/003,005
6. Meetings and Events
([NOTE] You can include the following in "6. Meetings and Events":
(1) Seminars and workshops by guest speaker(s)
(2) Seminars and workshops by guest speaker(s) and OIST faculty member(s)/unit member(s)
6.1 Seminars/Workshop
1. "Holotomography and artificial intelligence: label-free 3D imaging, classification, and inference of live cells and organoids"
https://groups.oist.jp/chaos/event/seminar-dryongkeun-park-holotomography-and-artificial-intelligence-label-free-3d-imaging
- Speaker: Dr.Yong Keun Park, Physics Department of KAIST (Korea Advanced Institute of Science and Technology), South Korea
- Date: May 29, 2023
- Venue: OIST Campus (Lab4, F01)
2. OIST Workshop "Manifolds in Nature"
- Date: February 26 - March 1, 2024
- Venue: OIST main campus (B250) and Seaside House
- Organizer: Gerald Pao
- Invited Speakers:
George Sugihara (Scripps Institution of Oceanography, UCSD)
Terrence Sejnowski (Salk Institute for Biological Studies)
Stanislav Smirnov (University of Geneva)
Misha Ahrens (Janelia Research Campus)
Lisa Li (University of Michigan)
Nuttida Rungratsameetaweemana (Columbia University)
Taro Toyoizumi (RIKEN CBS)
Yair Daon (Tel Aviv University)
Richard Gao (University of Tübingen)
Hiroaki Natsukawa (Osaka Seikei University)
Tatyana Sharpee (Salk Institute for Biological Studies)
Stephan Munch (NOAA Fisheries / University of California, Santa Cruz)
Tim Sauer (George Mason University)
6.2 Events
1. Title:Okinawa Computational Neuroscience course
- Date: June 23, 2023
- Venue: OIST Campus
Gerald Pao taught the “Neural activity manifolds” module (3 hrs of lecture module and follow up discussions with students and lab tour)
2. Hosted TSVP Coffee Chat with Stanislav Smirnov (Fields Medal 2010)
- Date: March 4, 2024
- Venue: OIST Campus (Lab 5 Atrium, Level D, East)
- Event page: https://groups.oist.jp/tsvp/event/tsvp-coffee-chat-stanislav-smirnov-fields-medal-2010
7. Other
Nothing to report.